DISCLAIMER: The idea of doing Cover Letter or even Resume with AI does not obviously start with me. A lot of people have done this before (very successfully) and have built websites and even companies from the idea. This is just a tutorial on how to build your own Cover Letter AI Generator App using Python and a few lines of code. All the code you will say in this blog post can be found in my public Github folder. Enjoy. 🙂
Pep Guardiola is a (very successful) Manchester City football coach. During Barcelona’s Leo Messi years, he invented a way of playing football known as “Tiki-Taka”. This means that as soon as you receive the ball, you pass the ball, immediately, without even controlling it. You can pass the ball 30–40 times before scoring a goal.
More than a decade later, we can see how the way of playing football made Guardiola and his Barcelona famous is gone. If you look at a Manchester City match, they take the ball and immediately look for the striker or the winger. You only need a few, vertical passes, immediately looking for the opportunity. It is more predictable, but you do it so many times that you will eventually find the space to hit the target.
I think that the job market has somehow gone in the same direction.
Before you had the opportunity to go to the company, hand in your resume, talk to them, be around them, schedule an interview, and actively talk to people. You would spend weeks preparing for that trip, polishing your resume, and reviewing questions and answers.
For many, this old-fashioned strategy still works, and I believe it. If you have a good networking opportunity, or the right time and place, the handing the resume thing works very well. We love the human connection, and it is very effective to actually know someone.
It is important to consider that there is a whole other approach as well. Companies like LinkedIn, Indeed, and even in general the internet completely changed the game. You can send so many resumes to so many companies and find a job out of statistics. AI is changing this game a little bit further. There are a lot of AI tools to tailor your resume for the specific company, make your resume more impressive, or build the job specific cover letter. There are indeed many companies that sell this kind of services to people that are looking for jobs.
Now, believe me, I have got nothing against these companies, at all, but the AI that they are using it’s not really “their AI”. What I mean by that is that if you use ChatGPT, Gemini, or the super new DeepSeek to do the exact task you will very likely not get a worse response than the (paid) tool that you are using on their website. You are really paying for the “commodity” of having a backend API that does what we would have to do through ChatGPT. And that’s fair.
Nonetheless, I want to show you that it is indeed very simple and cheap to make your own “resume assistant” using Large Language Models. In particular, I want to focus on cover letters. You give me your resume and the job description, and I give you your cover letter you can copy and paste to LinkedIn, Indeed, or your email.
In one image, it will look like this:
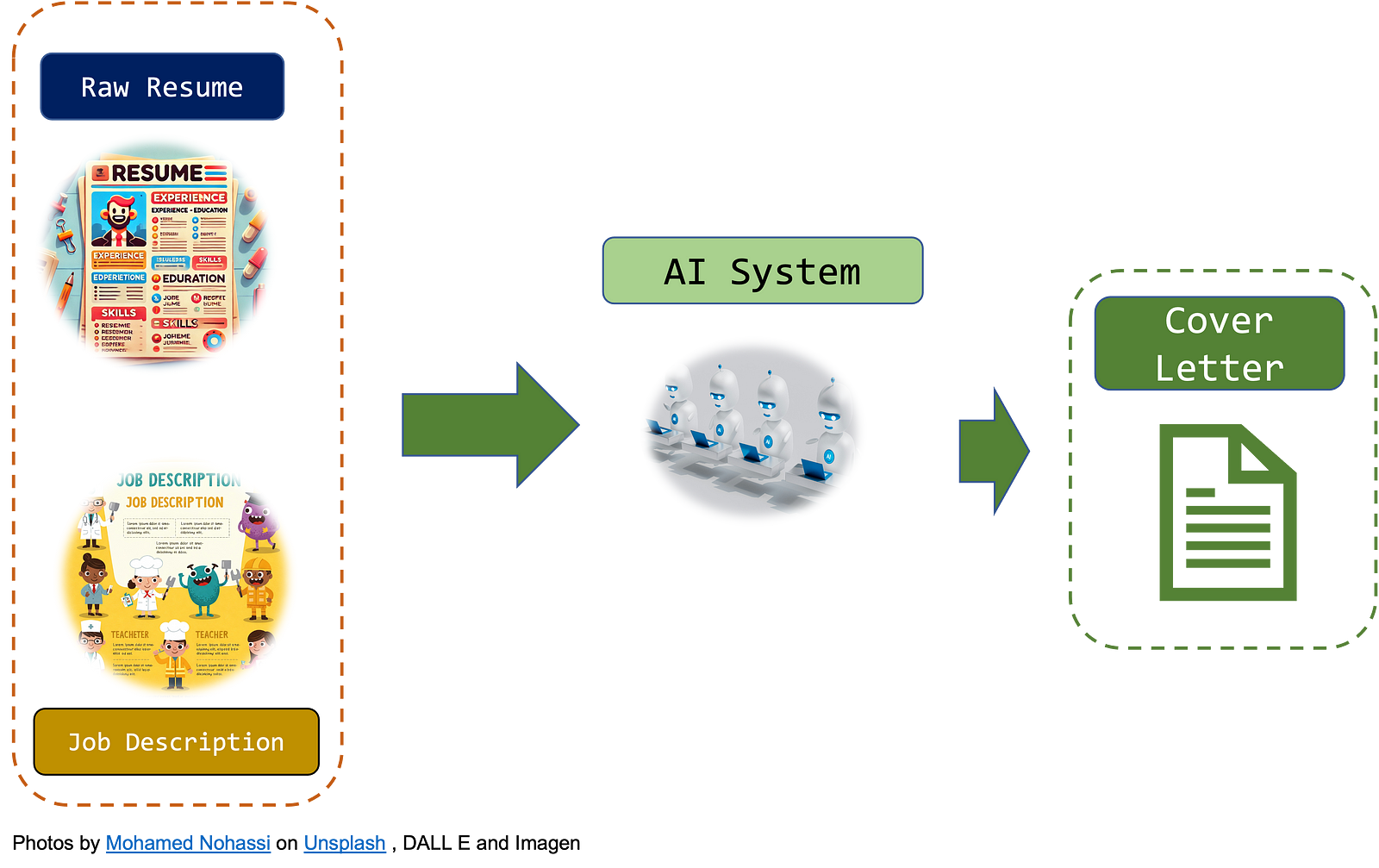
Now, Large Language Models (LLMs) are specific AI models that produce text. More specifically, they are HUGE Machine Learning models (even the small ones are very big).
This means that building your own LLM or training one from scratch is very, very expensive. We won’t do anything like that. We will use a perfectly working LLM and we will smartly instruct it to perform our task. More specifically, we will do that in Python and using some APIs. Formally, it is a paid API. Nonetheless, since I started the whole project (with all the trial and error process) I spent less than 30 cents. You will likely spend 4 or 5 cents on it.
Additionally, we will make a working web app that will allow you to have your cover letter in a few clicks. It will be an effort of a couple hundred lines of code (with spaces 🙂).
To motivate you, here are screenshots of the final app:
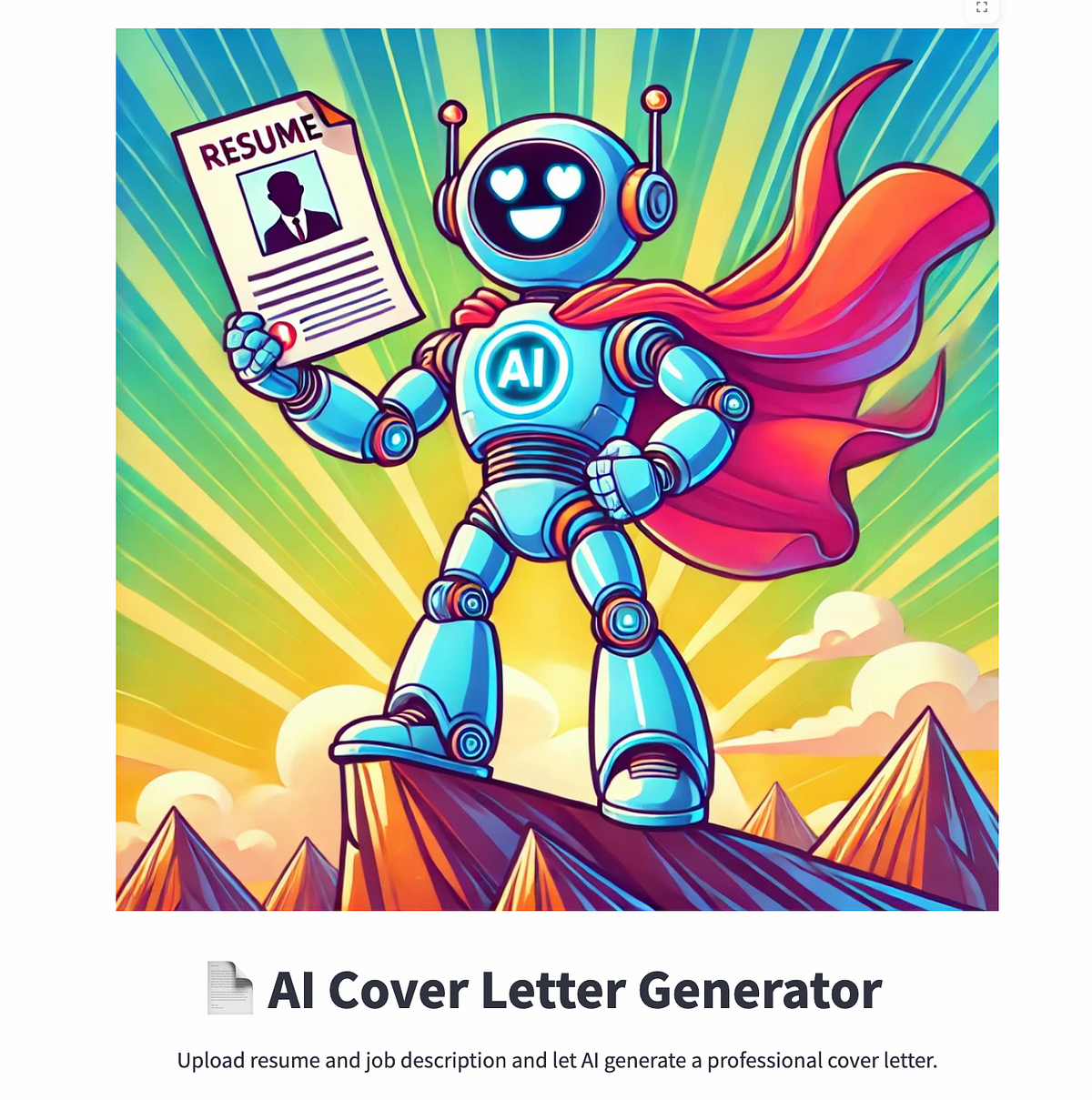
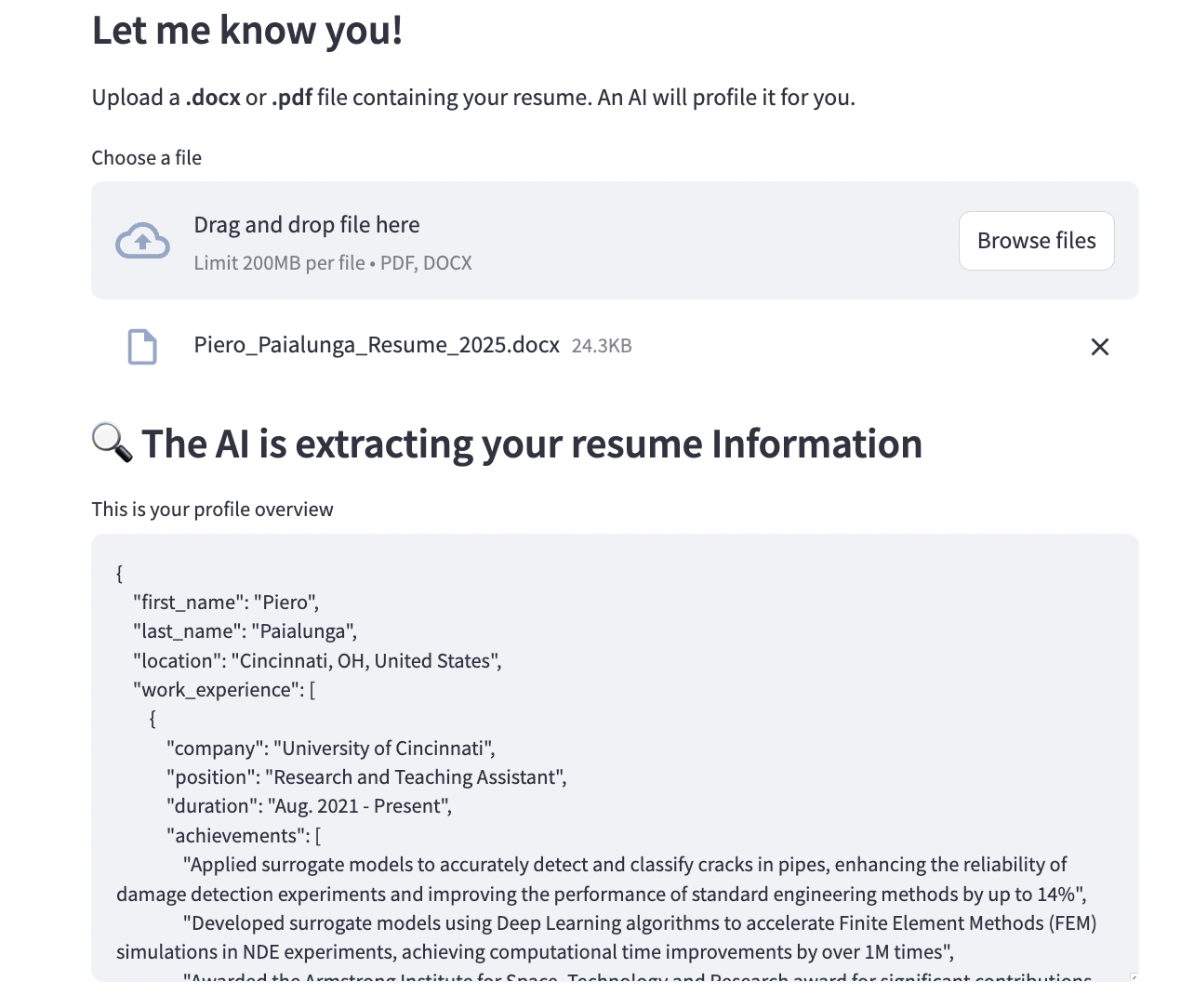
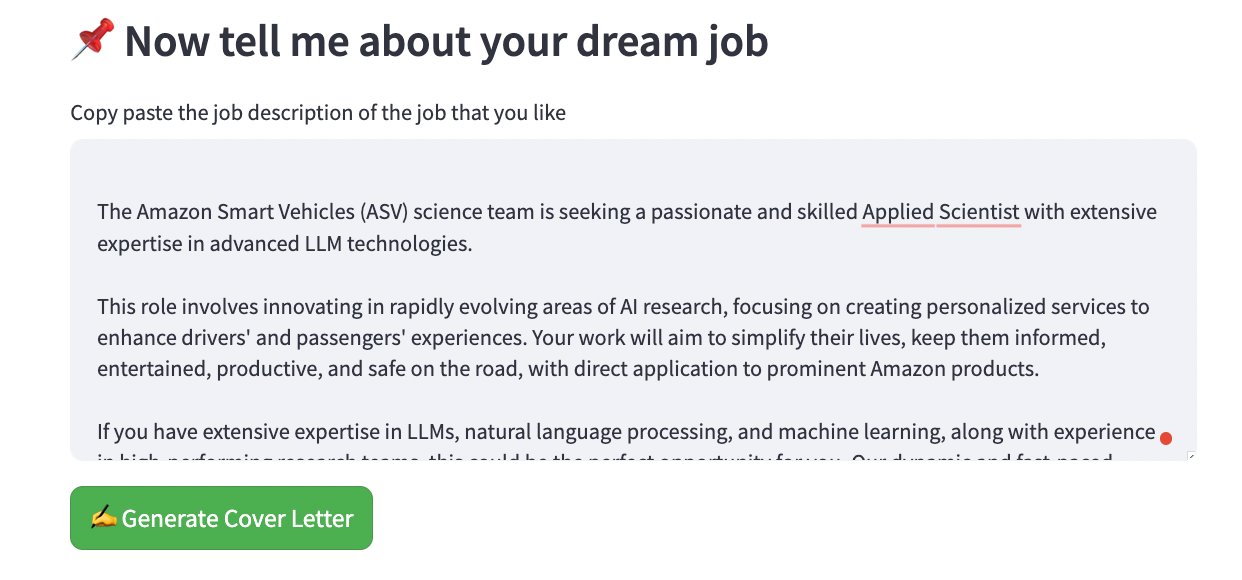
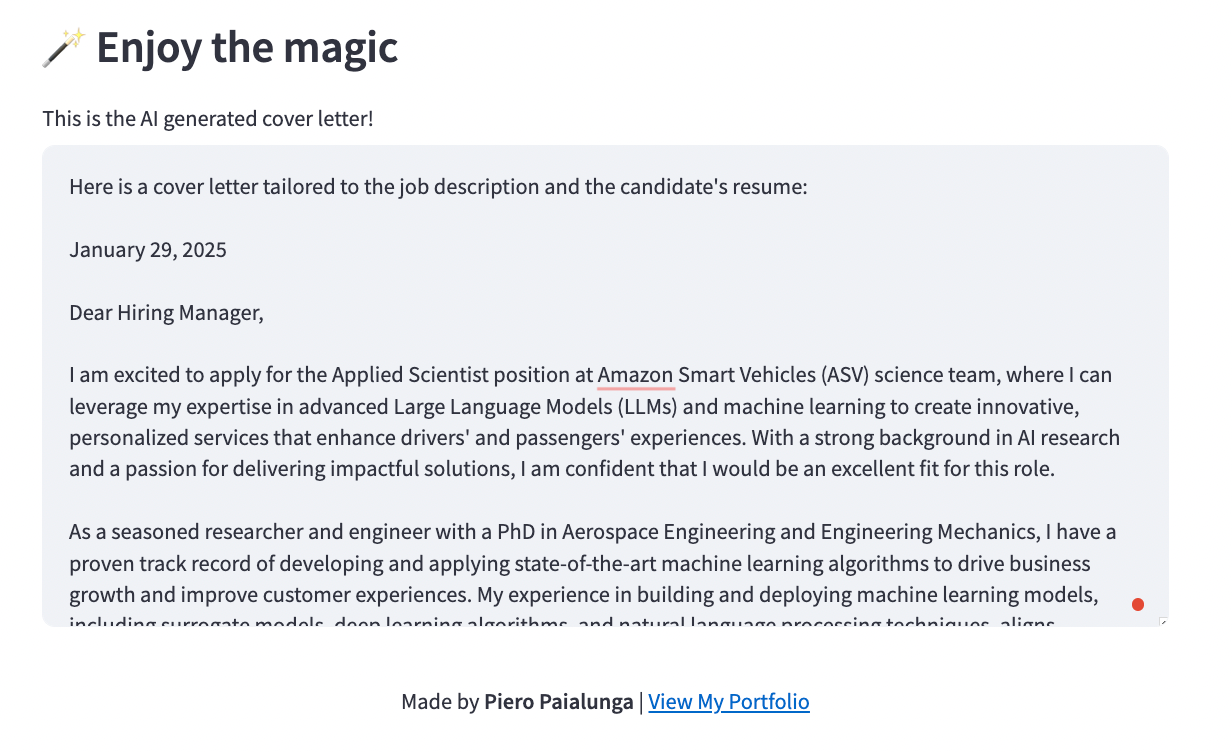
Pretty cool right? It took me less than 5 hours to build the whole thing from scratch. Believe me: it’s that simple. In this blog post, we will describe, in order:
- The LLM API Strategy. This part will help the reader understand what LLM Agents we are using and how we are connecting them.
- The LLM Object. This is the implementation of the LLM API strategy above using Python.
- The Web App and results. The LLM Object is then transferred into a web app using Streamlit. I’ll show you how to access it and some results.
I’ll try to be as specific as possible so that you have everything you need to make it yourself, but if this stuff gets too technical, feel free to skip to part 3 and just enjoy the sunset 🙃.
Let’s get started!
1. LLM API Strategy
This is the Machine Learning System Design part of this project, which I kept extremely light, because I wanted to maximize the readability of the whole approach (and because it honestly didn’t need to be more complicated than that).
We will use two APIs:
- A Document Parser LLM API will read the Resume and extract all the meaningful information. This information will be put in a .json file so that, in production, we will have the resume already processed and stored somewhere in our memory.
- A cover letter LLM API. This API will read the parsed resume (the output of the previous API) and the job description and it will output the Cover Letter.
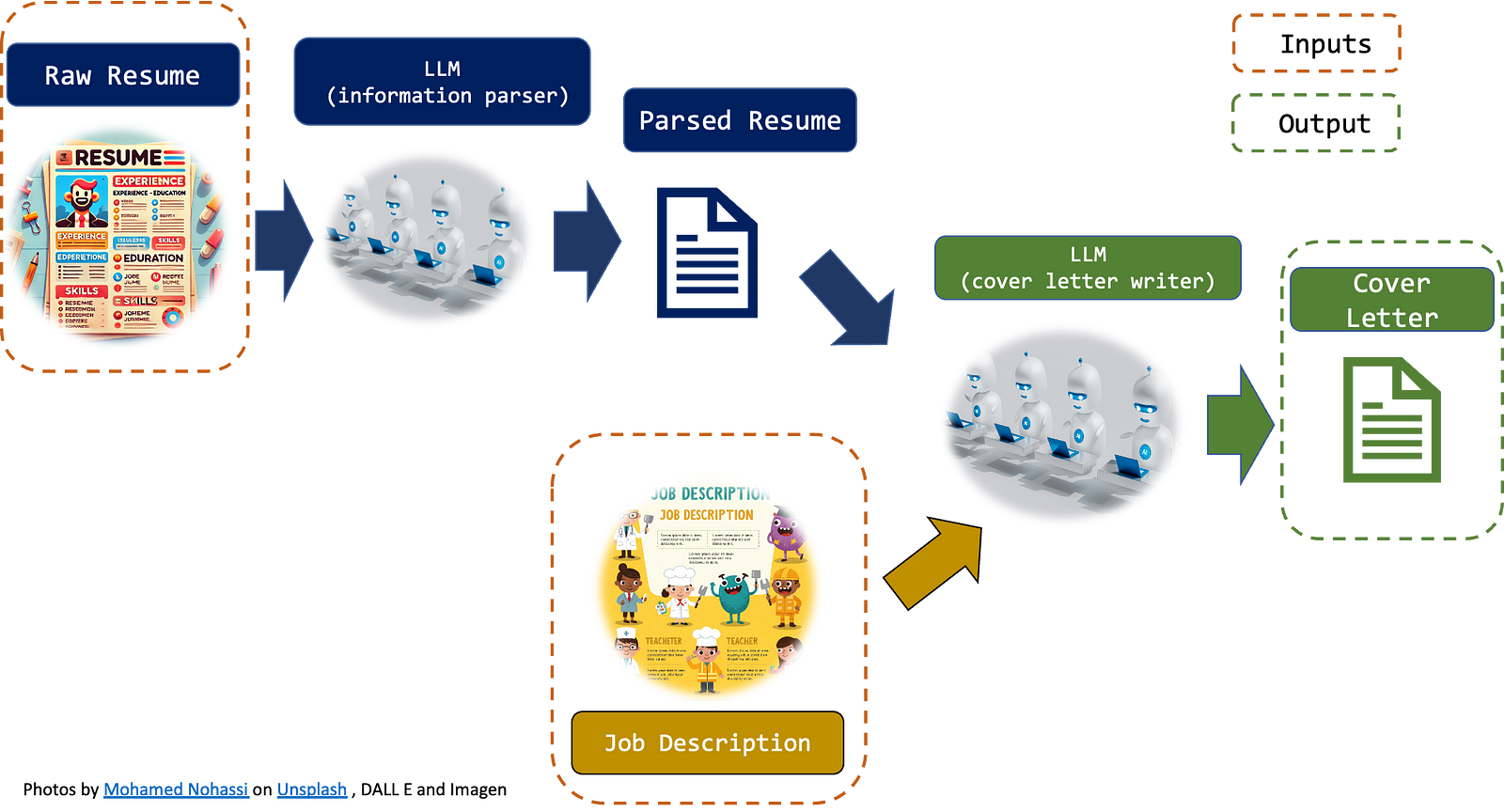
Two main points:
- What is the best LLM for this task? For text extraction and summarization, LLama or Gemma are known to be a reasonably cheap and efficient LLM. As we are going to use LLama for the summarization task, in order to keep consistency, we can adopt it for the other API as well. If you want to use another model, feel free to do so.
- How do we connect the APIs? There are a variety of ways you can do that. I decided to give it a try to Llama API. The documentation is not exactly extensive, but it works well and it allows you to play with many models. You will need to log in, buy some credit ($1 is more than sufficient for this task), and save your API key. Feel free to switch to another solution (like Hugging Face or Langchain) if you feel like it.
Ok, now that we know what to do, we just need to actually implement it in Python.
2. LLM Object
The first thing that we need is the actual LLM prompts. In the API, the prompts are usually passed using a dictionary. As they can be pretty long, and their structure is always similar, it makes sense to store them in .json files. We will read the JSON files and use them as inputs for the API call.
2.1 LLM Prompts
In this .json file, you will have the model (you can call whatever model you like) and the content which is the instruction for the LLM. Of course, the content key has a static part, which is the “instruction” and a “dynamic” part, which is the specific input of the API call. For example: this is the .json file for the first API, I called it resume_parser_api.json:
As you can see from the “content” there is the static call:
“You are a resume parser. You will extract information from this resume and put them in a .json file. The keys of your dictionary will be first_name, last_name, location, work_experience, school_experience, skills. In selecting the information, keep track of the most insightful.”
The keys I want to extract out of my “.json” files are:
[first_name, last_name, location, work_experience, school_experience, skills]
Feel free to add anything more information that you want to be “extracted” out of your resume, but remember that this is stuff that should matter only for your cover letter. The specific resume will be added after this text to form the full call/instruction. More on that later.
The order instruction is the cover_letter_api.json:
Now the instruction is this one:
“You are an expert in job hunting and a cover letter writer. Given a resume json file, the job description, and the date, write a cover letter for this candidate. Be persuasive and professional. Resume JSON: {resume_json} ; Job Description: {job_description}, Date: {date}”
As you can see, there are three placeholders: “Resume_json”, “job_description” and “date”. As before, these placeholders will then be replaced with the correct information to form the full prompt.
2.2 constants.py
I made a very small constants.py file with the path of the two .json prompt files and the API that you have to generate from LLamaApi (or really whatever API you are using). Modify this if you want to run the file locally.
2.3 file_loader.py
This file is a collection of “loaders” for your resume. Boring stuff but important.
2.4 cover_letter.py
The whole implementation of the LLM Strategy can be found in this object that I called CoverLetterAI. There it is:
I spent quite some time trying to make everything modular and easy to read. I also made a lot of comments to all the functions so you can see exactly what does what. How do we use this beast?
So the whole code runs in 5 simple lines. Like this:
from cover_letter import CoverLetterAI
cover_letter_AI = CoverLetterAI()
cover_letter_AI.read_candidate_data('path_to_your_resume_file')
cover_letter_AI.profile_candidate()
cover_letter_AI.add_job_description('Insert job description')
cover_letter_AI.write_cover_letter()
So in order:
- You call the CoverLetterAI object. It will be the star of the show
- You give me the path to your resume. It can be PDF or Word and I read your information and store them in a variable.
- You call profile_candidate(), and I run my first LLM. This process the candidate word info and creates the .json file we will use for the second LLM
- You give me the job_description and you add it to the system. Stored.
- You call write_cover_letter() and I run my second LLM that generates, given the job description and the resume .json file, the cover letter
3. Web App and Results
So that is really it. You saw all the technical details of this blog post in the previous paragraphs.
Just to be extra fancy and show you that it works, I also made it a web app, where you can just upload your resume, add your job description and click generate cover letter. This is the link and this is the code.
Now, the cover letters that are generated are scary good.
This is a random one:
February 1, 2025
Hiring Manager,
[Company I am intentionally blurring]I am thrilled to apply for the Distinguished AI Engineer position at [Company I am intentionally blurring], where I can leverage my passion for building responsible and scalable AI systems to revolutionize the banking industry. As a seasoned machine learning engineer and researcher with a strong background in physics and engineering, I am confident that my skills and experience align with the requirements of this role.
With a Ph.D. in Aerospace Engineering and Engineering Mechanics from the University of Cincinnati and a Master’s degree in Physics of Complex Systems and Big Data from the University of Rome Tor Vergata, I possess a unique blend of theoretical and practical knowledge. My experience in developing and deploying AI models, designing and implementing machine learning algorithms, and working with large datasets has equipped me with the skills to drive innovation in AI engineering.
As a Research and Teaching Assistant at the University of Cincinnati, I applied surrogate models to detect and classify cracks in pipes, achieving a 14% improvement in damage detection experiments. I also developed surrogate models using deep learning algorithms to accelerate Finite Element Methods (FEM) simulations, resulting in a 1M-fold reduction in computational time. My experience in teaching and creating courses in signal processing and image processing for teens interested in AI has honed my ability to communicate complex concepts effectively.
In my previous roles as a Machine Learning Engineer at Gen Nine, Inc., Apex Microdevices, and Accenture, I have successfully designed, developed, and deployed AI-powered solutions, including configuring mmWave radar and Jetson devices for data collection, implementing state-of-the-art point cloud algorithms, and leading the FastMRI project to accelerate MRI scan times. My expertise in programming languages such as Python, TensorFlow, PyTorch, and MATLAB, as well as my experience with cloud platforms like AWS, Docker, and Kubernetes, has enabled me to develop and deploy scalable AI solutions.
I am particularly drawn to [Company I am intentionally blurring] commitment to creating responsible and reliable AI systems that prioritize customer experience and simplicity. My passion for staying abreast of the latest AI research and my ability to judiciously apply novel techniques in production align with the company’s vision. I am excited about the opportunity to work with a cross-functional team of engineers, research scientists, and product managers to deliver AI-powered products that transform how [Company I am intentionally blurring] serves its customers.
In addition to my technical skills and experience, I possess excellent communication and presentation skills, which have been demonstrated through my technical writing experience at Towards Data Science, where I have written comprehensive articles on machine learning and data science, reaching a broad audience of 50k+ monthly viewers.
Thank you for considering my application. I am eager to discuss how my skills and experience can contribute to the success of the [Company I am intentionally blurring] and [Company I am intentionally blurring]’s mission to bring humanity and simplicity to banking through AI. I am confident that my passion for AI, my technical expertise, and my ability to work collaboratively will make me a valuable asset to your team.
Sincerely,
Piero Paialunga
They look just like I would write them for a specific job description. That being said, in 2025, you need to be careful because hiring managers do know that you are using AI to write them and the “computer tone” is pretty easy to spot (e.g. words like “eager” are very ChatGPT-ish lol). For this reason, I’d like to say to use these tools wisely. Sure, you can build your “template” with them, but be sure to add your personal touch, otherwise your cover letter will be exactly like the other thousands of cover letters that the other applicants are sending in.
This is the code to build the web app.
4. Conclusions
In this blog article, we discovered how to use LLM to convert your resume and job description into a specific cover letter. These are the points we touched:
- The use of AI in job hunting. In the first chapter we discussed how job hunting is now completely revolutionized by AI.
- Large Language Models idea. It is important to design the LLM APIs wisely. We did that in the second paragraph
- LLM API implementation. We used Python to implement the LLM APIs organically and efficiently
- The Web App. We used streamlit to build a Web App API to display the power of this approach.
- Limits of this approach. I think that AI generated cover letters are indeed very good. They are on point, professional and well crafted. Nonetheless, if everyone starts using AI to build cover letters, they all really look the same, or at least they all have the same tone, which is not great. Something to think about.
5. References and other brilliant implementations
I feel that is just fair to mention a lot of brilliant people that have had this idea before me and have made this public and available for anyone. This is only a few of them I found online.
Cover Letter Craft by Balaji Kesavan is a Streamlit app that implements a very similar idea of crafting the cover letter using AI. What we do different from that app is that we extract the resume directly from the word or PDF, while his app requires copy-pasteing. That being said, I think the guy is incredibly talented and very creative and I recommend giving a look to his portoflio.
Randy Pettus has a similar idea as well. The difference between his approach and the one proposed in this tutorial is that he is very specific in the information, asking questions like “current hiring manager” and the temperature of the model. It’s very interesting (and smart) that you can clearly see the way he is thinking of Cover Letters to guide the AI to build it the way he likes them. Highly recommended.
Juan Esteban Cepeda does a very good job in his app as well. You can also tell that he was working on making it bigger than a simple streamlit add because he added the link to his company and a bunch of reviews by users. Great job and great hustle. 🙂
6. About me!
Thank you again for your time. It means a lot ❤
My name is Piero Paialunga and I’m this guy here:
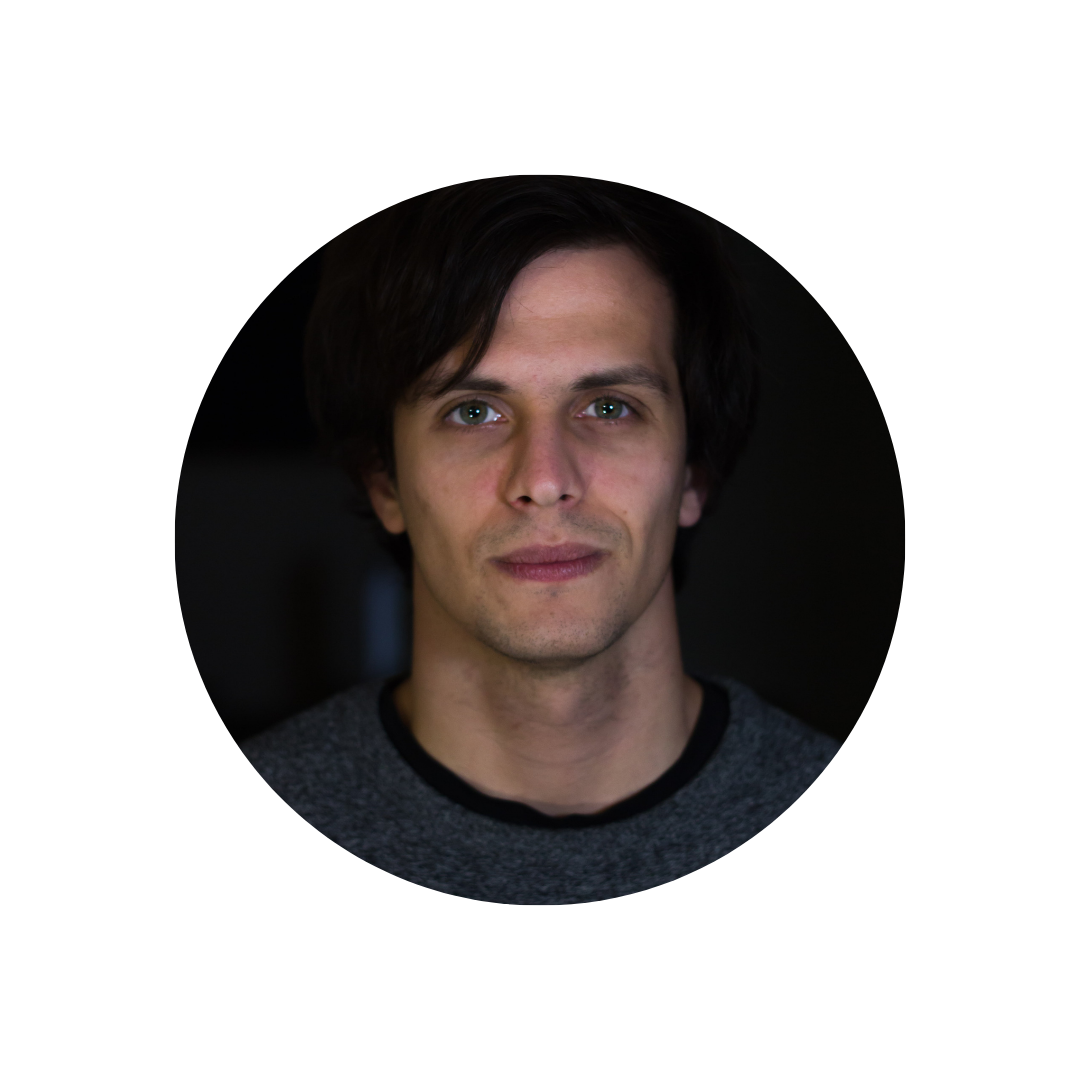
I am a Ph.D. candidate at the University of Cincinnati Aerospace Engineering Department and a Machine Learning Engineer for Gen Nine. I talk about AI, and Machine Learning in my blog posts and on Linkedin. If you liked the article and want to know more about machine learning and follow my studies you can:
A. Follow me on Linkedin, where I publish all my stories
B. Subscribe to my newsletter. It will keep you updated about new stories and give you the chance to text me to receive all the corrections or doubts you may have.
C. Become a referred member, so you won’t have any “maximum number of stories for the month” and you can read whatever I (and thousands of other Machine Learning and Data Science top writers) write about the newest technology available.
D. Want to work with me? Check my rates and projects on Upwork!
If you want to ask me questions or start a collaboration, leave a message here or on Linkedin: