As a data scientist, you know that effectively communicating your insights is as important as the insights themselves.
But how do you communicate over 3D data?
I can bet most of us have been there: you spend days, weeks, maybe even months meticulously collecting and processing 3D data. Then comes the moment to share your findings, whether it’s with clients, colleagues, or the broader scientific community. You throw together a few static screenshots, but they just don’t capture the essence of your work. The subtle details, the spatial relationships, the sheer scale of the data—it all gets lost in translation.

Or maybe you’ve tried using specialized 3D visualization software. But when your client uses it, they struggle with clunky interfaces, steep learning curves, and restrictive licensing.
What should be a smooth, intuitive process becomes a frustrating exercise in technical acrobatics. It’s an all-too-common scenario: the brilliance of your 3D data is trapped behind a wall of technical barriers.
This highlights a common issue: the need to create shareable content that can be opened by anyone, i.e., that does not demand specific 3D data science skills.
Think about it: what is the most used way to share visual information? Images.
But how can we convey the 3D information from a simple 2D image?
Well, let us use “first principle thinking”: let us create shareable content stacking multiple 2D views, such as GIFs or MP4s, from raw point clouds.
The bread of magic to generate GIF and MP4. © F. Poux
This process is critical for presentations, reports, and general communication. But generating GIFs and MP4s from 3D data can be complex and time-consuming. I’ve often found myself wrestling with the challenge of quickly generating rotating GIF or MP4 files from a 3D point cloud, a task that seemed simple enough but often spiraled into a time-consuming ordeal.
Current workflows might lack efficiency and ease of use, and a streamlined process can save time and improve data presentation.
Let me share a solution that involves leveraging Python and specific libraries to automate the creation of GIFs and MP4s from point clouds (or any 3D dataset such as a mesh or a CAD model).
Think about it. You’ve spent hours meticulously collecting and processing this 3D data. Now, you need to present it in a compelling way for a presentation or a report. But how can we be sure it can be integrated into a SaaS solution where it is triggered on upload? You try to create a dynamic visualization to showcase a critical feature or insight, and yet you’re stuck manually capturing frames and stitching them together. How can we automate this process to seamlessly integrate it into your existing systems?
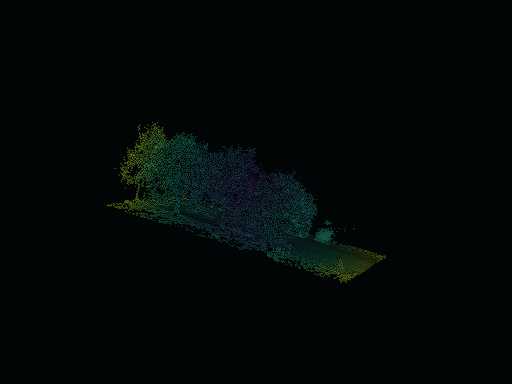
If you are new to my (3D) writing world, welcome! We are going on an exciting adventure that will allow you to master an essential 3D Python skill. Before diving, I like to establish a clear scenario, the mission brief.
Once the scene is laid out, we embark on the Python journey. Everything is given. You will see Tips (🦚Notes and 🌱Growing) to help you get the most out of this article. Thanks to the 3D Geodata Academy for supporting the endeavor.
The Mission 🎯
You are working for a new engineering firm, “Geospatial Dynamics,” which wants to showcase its cutting-edge LiDAR scanning services. Instead of sending clients static point cloud images, you propose to use a new tool, which is a Python script, to generate dynamic rotating GIFs of project sites.
After doing so market research, you found that this can immediately elevate their proposals, resulting in a 20% higher project approval rate. That’s the power of visual storytelling.

On top, you can even imagine a more compelling scenario, where “GeoSpatial Dynamics” is able to process point clouds massively and then generate MP4 videos that are sent to potential clients. This way, you lower the churn and make the brand more memorable.
With that in mind, we can start designing a robust framework to answer our mission’s goal.
The Framework
I remember a project where I had to show a detailed architectural scan to a group of investors. The usual still images just could not capture the fine details. I desperately needed a way to create a rotating GIF to convey the full scope of the design. That is why I’m excited to introduce this Cloud2Gif Python solution. With this, you’ll be able to easily generate shareable visualizations for presentations, reports, and communication.
The framework I propose is straightforward yet effective. It takes raw 3D data, processes it using Python and the PyVista library, generates a series of frames, and stitches them together to create a GIF or MP4 video. The high-level workflow includes:

1. Loading the 3D data (mesh with texture).
2. Loading a 3D Point Cloud
3. Setting up the visualization environment.
4. Generating a GIF
4.1. Defining a camera orbit path around the data.
4.2. Rendering frames from different viewpoints along the path.
4.3. Encoding the frames into a GIF or
5. Generating an orbital MP4
6. Creating a Function
7. Testing with multiple datasets
This streamlined process allows for easy customization and integration into existing workflows. The key advantage here is the simplicity of the approach. By leveraging the basic principles of 3D data rendering, a very efficient and self-contained script can be put together and deployed on any system as long as Python is installed.
This makes it compatible with various edge computing solutions and allows for easy integration with sensor-heavy systems. The goal is to generate a GIF and an MP4 from a 3D data set. The process is simple, requiring a 3D data set, a bit of magic (the code), and the output as GIF and MP4 files.

Now, what are the tools and libraries that we will need for this endeavor?
1. Setup Guide: The Libraries, Tools and Data

For this project, we primarily use the following two Python libraries:
- NumPy: The cornerstone of numerical computing in Python. Without it, I would have to deal with every vertex (point) in a very inefficient way. NumPy Official Website
- pyvista: A high-level interface to the Visualization Toolkit (VTK). PyVista enables me to easily visualize and interact with 3D data. It handles rendering, camera control, and exporting frames. PyVista Official Website

These libraries provide all the necessary tools to handle data processing, visualization, and output generation. This set of libraries was carefully chosen so that a minimal amount of external dependencies is present, which improves sustainability and makes it easily deployable on any system.
Let me share the details of the environment as well as the data preparation setup.
Quick Environment Setup Guide
Let me provide very brief details on how to set up your environment.
Step 1: Install Miniconda
Four simple steps to get a working Miniconda version:
- Visit: https://docs.conda.io/projects/miniconda/en/latest/
- Download the “installer file” for your Operating System (Let it be Windows, MacOS or a Linux distribution)
- Run the installer
- Open terminal/command prompt and verify with: conda — version

Step 2: Create a new environment
You can run the following code in your terminal
conda create -n pyvista_env python=3.10
conda activate pyvista_env
Step 3: Install required packages
For this, you can leverage pip as follows:
pip install numpy
pip install pyvista
Step 4: Test the installation
If you want to test your installation, type python in your terminal and run the following lines:
import numpy as np
import pyvista as pv
print(f”PyVista version: {pv.__version__}”)
This should return the pyvista
version. Do not forget to exit Python from your terminal afterward (Ctrl+C
).
🦚 Note: Here are some common issues and workarounds:
- If PyVista doesn’t show a 3D window:
pip install vtk
- If environment activation fails: Restart the terminal
- If data loading fails: Check file format compatibility (
PLY
,LAS
,LAZ
supported)
Beautiful, at this stage, your environment is ready. Now, let me share some quick ways to get your hands on 3D datasets.
Data Preparation for 3D Visualization
At the end of the article, I share with you the datasets as well as the code. However, in order to ensure you are fully independent, here are three reliable sources I regularly use to get my hands on point cloud data:

The USGS 3DEP LiDAR Point Cloud Downloads
OpenTopography
ETH Zurich’s PCD Repository
For quick testing, you can also use PyVista’s built-in example data:
# Load sample data
from pyvista import examples
terrain = examples.download_crater_topo()
terrain.plot()
🦚 Note: Remember to always check the data license and attribution requirements when using public datasets.
Finally, to ensure a complete setup, below is a typical expected folder structure:
project_folder/
├── environment.yml
├── data/
│ └── pointcloud.ply
└── scripts/
└── gifmaker.py
Beautiful, we can now jump right onto the first stage: loading and visualizing textured mesh data.
2. Loading and Visualizing Textured Mesh Data
One first critical step is properly loading and rendering 3D data. In my research laboratory, I have found that PyVista provides an excellent foundation for handling complex 3D visualization tasks.

Here’s how you can approach this fundamental step:
import numpy as np
import pyvista as pv
mesh = pv.examples.load_globe()
texture = pv.examples.load_globe_texture()
pl = pv.Plotter()
pl.add_mesh(mesh, texture=texture, smooth_shading=True)
pl.show()
This code snippet loads a textured globe mesh, but the principles apply to any textured 3D model.

Let me discuss and speak a bit about the smooth_shading parameter. It’s a tiny element that renders the surfaces more continuous (as opposed to faceted), which, in the case of spherical objects, improves the visual impact.
Now, this is just a starter for 3D mesh data. This means that we deal with surfaces that join points together. But what if we want to work solely with point-based representations?
In that scenario, we have to consider shifting our data processing approach to propose solutions to the unique visual challenges attached to point cloud datasets.
3. Point Cloud Data Integration
Point cloud visualization demands extra attention to detail. In particular, adjusting the point density and the way we represent points on the screen has a noticeable impact.

Let us use a PLY file for testing (see the end of the article for resources).

You can load a point cloud pv.read and create scalar fields for better visualization (such as using a scalar field based on the height or extent around the center of the point cloud).
In my work with LiDAR datasets, I’ve developed a simple, systematic approach to point cloud loading and initial visualization:
cloud = pv.read('street_sample.ply')
scalars = np.linalg.norm(cloud.points - cloud.center, axis=1)
pl = pv.Plotter()
pl.add_mesh(cloud)
pl.show()
The scalar computation here is particularly important. By calculating the distance from each point to the cloud’s center, we create a basis for color-coding that helps convey depth and structure in our visualizations. This becomes especially valuable when dealing with large-scale point clouds where spatial relationships might not be immediately apparent.
Moving from basic visualization to creating engaging animations requires careful consideration of the visualization environment. Let’s explore how to optimize these settings for the best possible results.
4. Optimizing the Visualization Environment
The visual impact of our animations heavily depends on the visualization environment settings.

Through extensive testing, I’ve identified key parameters that consistently produce professional-quality results:
pl = pv.Plotter(off_screen=False)
pl.add_mesh(
cloud,
style='points',
render_points_as_spheres=True,
emissive=False,
color='#fff7c2',
scalars=scalars,
opacity=1,
point_size=8.0,
show_scalar_bar=False
)
pl.add_text('test', color='b')
pl.background_color = 'k'
pl.enable_eye_dome_lighting()
pl.show()
As you can see, the plotter is initialized off_screen=False to render directly to the screen. The point cloud is then added to the plotter with specified styling. The style=’points’ parameter ensures that the point cloud is rendered as individual points. The scalars=’scalars’ argument uses the previously computed scalar field for coloring, while point_size sets the size of the points, and opacity adjusts the transparency. A base color is also set.
🦚 Note: In my experience, rendering points as spheres significantly improves the depth perception in the final generated animation. You can also combine this by using the eye_dome_lighting feature. This algorithm adds another layer of depth cues through some sort of normal-based shading, which makes the structure of point clouds more apparent.
You can play around with the various parameters until you obtain a rendering that is satisfying for your applications. Then, I propose that we move to creating the animated GIFs.
A GIF of the point cloud. © F. Poux
5. Creating Animated GIFs
At this stage, our aim is to generate a series of renderings by varying the viewpoint from which we generate these.

This means that we need to design a camera path that is sound, from which we can generate frame rendering.
This means that to generate our GIF, we must first create an orbiting path for the camera around the point cloud. Then, we can sample the path at regular intervals and capture frames from different viewpoints.
These frames can then be used to create the GIF. Here are the steps:

- I change to
off-screen
rendering - I take the cloud length parameters to set the camera
- I create a path
- I create a loop that takes a point of this pass
Which translates into the following:
pl = pv.Plotter(off_screen=True, image_scale=2)
pl.add_mesh(
cloud,
style='points',
render_points_as_spheres=True,
emissive=False,
color='#fff7c2',
scalars=scalars,
opacity=1,
point_size=5.0,
show_scalar_bar=False
)
pl.background_color = 'k'
pl.enable_eye_dome_lighting()
pl.show(auto_close=False)
viewup = [0, 0, 1]
path = pl.generate_orbital_path(n_points=40, shift=cloud.length, viewup=viewup, factor=3.0)
pl.open_gif("orbit_cloud_2.gif")
pl.orbit_on_path(path, write_frames=True, viewup=viewup)
pl.close()
As you can see, an orbital path is created around the point cloud using pl.generate_orbital_path()
. The path’s radius is determined by cloud_length, the center is set to the center of the point cloud, and the normal vector is set to [0, 0, 1]
, indicating that the circle lies in the XY
plane.
From there, we can enter a loop to generate individual frames for the GIF (the camera’s focal point is set to the center of the point cloud).
The image_scale
parameter deserves special attention—it determines the resolution of our output.
I’ve found that a value of 2 provides a good balance between the perceived quality and the file size. Also, the
viewup
vector is crucial for maintaining proper orientation throughout the animation. You can experiment with its value if you want a rotation following a non-horizontal plane.
This results in a GIF that you can use to communicate very easily.
Another synthetic point cloud generated GIF. © F. Poux
But we can push one extra stage: creating an MP4 video. This can be useful if you want to obtain higher-quality animations with smaller file sizes as compared to GIFs (which are not as compressed).
6. High-Quality MP4 Video Generation
The generation of an MP4 video follows the exact same principles as we used to generate our GIF.

Therefore, let me get straight to the point. To generate an MP4 file from any point cloud, we can reason in four stages:

- Gather your configurations over the parameters that best suit you.
- Create an orbital path the same way you did with GIFs
- Instead of using the
open_gif function
, let us use itopen_movie
to write a “movie” type file. - We orbit on the path and write the frames, similarly to our GIF method.
🦚 Note: Don’t forget to use your proper configuration in the definition of the path.
This is what the end result looks like with code:
pl = pv.Plotter(off_screen=True, image_scale=1)
pl.add_mesh(
cloud,
style='points_gaussian',
render_points_as_spheres=True,
emissive=True,
color='#fff7c2',
scalars=scalars,
opacity=0.15,
point_size=5.0,
show_scalar_bar=False
)
pl.background_color = 'k'
pl.show(auto_close=False)
viewup = [0.2, 0.2, 1]
path = pl.generate_orbital_path(n_points=40, shift=cloud.length, viewup=viewup, factor=3.0)
pl.open_movie("orbit_cloud.mp4")
pl.orbit_on_path(path, write_frames=True)
pl.close()
Notice the use of points_gaussian
style and adjusted opacity—these settings provide interesting visual quality in video format, particularly for dense point clouds.
And now, what about streamlining the process?
7. Streamlining the Process with a Custom Function

To make this process more efficient and reproducible, I’ve developed a function that encapsulates all these steps:
def cloudgify(input_path):
cloud = pv.read(input_path)
scalars = np.linalg.norm(cloud.points - cloud.center, axis=1)
pl = pv.Plotter(off_screen=True, image_scale=1)
pl.add_mesh(
cloud,
style='Points',
render_points_as_spheres=True,
emissive=False,
color='#fff7c2',
scalars=scalars,
opacity=0.65,
point_size=5.0,
show_scalar_bar=False
)
pl.background_color = 'k'
pl.enable_eye_dome_lighting()
pl.show(auto_close=False)
viewup = [0, 0, 1]
path = pl.generate_orbital_path(n_points=40, shift=cloud.length, viewup=viewup, factor=3.0)
pl.open_gif(input_path.split('.')[0]+'.gif')
pl.orbit_on_path(path, write_frames=True, viewup=viewup)
pl.close()
path = pl.generate_orbital_path(n_points=100, shift=cloud.length, viewup=viewup, factor=3.0)
pl.open_movie(input_path.split('.')[0]+'.mp4')
pl.orbit_on_path(path, write_frames=True)
pl.close()
return
🦚 Note: This function standardizes our visualization process while maintaining flexibility through its parameters. It incorporates several optimizations I’ve developed through extensive testing. Note the different n_points values for GIF (40) and MP4 (100)—this balances file size and smoothness appropriately for each format. The automatic filename generation
split(‘.’)[0]
ensures consistent output naming.
And what better than to test our new creation on multiple datasets?
8. Batch Processing Multiple Datasets

Finally, we can apply our function to multiple datasets:
dataset_paths= ["lixel_indoor.ply", "NAAVIS_EXTERIOR.ply", "pcd_synthetic.ply", "the_adas_lidar.ply"]
for pcd in dataset_paths:
cloudgify(pcd)
This approach can be remarkably efficient when processing large datasets made of several files. Indeed, if your parametrization is sound, you can maintain consistent 3D visualization across all outputs.
🌱 Growing: I am a big fan of 0% supervision to create 100% automatic systems. This means that if you want to push the experiments even more, I suggest investigating ways to automatically infer the parameters based on the data, i.e., data-driven heuristics. Here is an example of a paper I wrote a couple of years down the line that focuses on such an approach for unsupervised segmentation (Automation in Construction, 2022)
A Little Discussion
Alright, you know my tendency to push innovation. While relatively simple, this Cloud2Gif solution has direct applications that can help you propose better experiences. Three of them come to mind, which I leverage on a weekly basis:

- Interactive Data Profiling and Exploration: By generating GIFs of complex simulation results, I can profile my results at scale very quickly. Indeed, the qualitative analysis is thus a matter of slicing a sheet filled with metadata and GIFs to check if the results are on par with my metrics. This is very handy
- Educational Materials: I often use this script to generate engaging visuals for my online courses and tutorials, enhancing the learning experience for the professionals and students that go through it. This is especially true now that most material is found online, where we can leverage the capacity of browsers to play animations.
- Real-time Monitoring Systems: I worked on integrating this script into a real-time monitoring system to generate visual alerts based on sensor data. This is especially relevant for sensor-heavy systems, where it can be difficult to extract meaning from the point cloud representation manually. Especially when conceiving 3D Capture Systems, leveraging SLAM or other techniques, it can be helpful to get a feedback loop in real-time to ensure a cohesive registration.
However, when we consider the broader research landscape and the pressing needs of the 3D data community, the real value proposition of this approach becomes evident. Scientific research is increasingly interdisciplinary, and communication is key. We need tools that enable researchers from diverse backgrounds to understand and share complex 3D data easily.
The Cloud2Gif script is self-contained and requires minimal external dependencies. This makes it ideally suited for deployment on resource-constrained edge devices. And this may be the top application that I worked on, leveraging such a straightforward approach.
As a little digression, I saw the positive impact of the script in two scenarios. First, I designed an environmental monitoring system for diseases in farmland crops. This was a 3D project, and I could include the generation of visual alerts (with an MP4 file) based on the real-time LiDAR sensor data. A great project!
In another context, I wanted to provide visual feedback to on-site technicians using a SLAM-equipped system for mapping purposes. I integrated the process to generate a GIF every 30 seconds that showed the current state of data registration. It was a great way to ensure consistent data capture. This actually allowed us to reconstruct complex environments with better consistency in managing our data drift.
Conclusion
Today, I walked through a simple yet powerful Python script to transform 3D data into dynamic GIFs and MP4 videos. This script, combined with libraries like NumPy and PyVista, allows us to create engaging visuals for various applications, from presentations to research and educational materials.
The key here is accessibility: the script is easily deployable and customizable, providing an immediate way of transforming complex data into an accessible format. This Cloud2Gif script is an excellent piece for your application if you need to share, assess, or get quick visual feedback within data acquisition situations.
What is next?
Well, if you feel up for a challenge, you can create a simple web application that allows users to upload point clouds, trigger the video generation process, and download the resulting GIF or MP4 file.
This, in a similar manner as shown here:
In addition to Flask, you can also create a simple web application that can be deployed on Amazon Web Services so that it is scalable and easily accessible to anyone, with minimal maintenance.
These are skills that you develop through the Segmentor OS Program at the 3D Geodata Academy.
About the author
Florent Poux, Ph.D. is a Scientific and Course Director focused on educating engineers on leveraging AI and 3D Data Science. He leads research teams and teaches 3D Computer Vision at various universities. His current aim is to ensure humans are correctly equipped with the knowledge and skills to tackle 3D challenges for impactful innovations.
Resources
- 🏆Awards: Jack Dangermond Award
- 📕Book: 3D Data Science with Python
- 📜Research: 3D Smart Point Cloud (Thesis)
- 🎓Courses: 3D Geodata Academy Catalog
- 💻Code: Florent’s Github Repository
- 💌3D Tech Digest: Weekly Newsletter