We all know what it means, colloquially, to google something. You pop a few relevant words in a search box and in return get a list of blue links to the most relevant results. Maybe some quick explanations up top. Maybe some maps or sports scores or a video. But fundamentally, it’s just fetching information that’s already out there on the internet and showing it to you, in some sort of structured way.
But all that is up for grabs. We are at a new inflection point.
The biggest change to the way search engines have delivered information to us since the 1990s is happening right now. No more keyword searching. No more sorting through links to click. Instead, we’re entering an era of conversational search. Which means instead of keywords, you use real questions, expressed in natural language. And instead of links, you’ll increasingly be met with answers, written by generative AI and based on live information from all across the internet, delivered the same way.
Of course, Google—the company that has defined search for the past 25 years—is trying to be out front on this. In May of 2023, it began testing AI-generated responses to search queries, using its large language model (LLM) to deliver the kinds of answers you might expect from an expert source or trusted friend. It calls these AI Overviews. Google CEO Sundar Pichai described this to MIT Technology Review as “one of the most positive changes we’ve done to search in a long, long time.”
AI Overviews fundamentally change the kinds of queries Google can address. You can now ask it things like “I’m going to Japan for one week next month. I’ll be staying in Tokyo but would like to take some day trips. Are there any festivals happening nearby? How will the surfing be in Kamakura? Are there any good bands playing?” And you’ll get an answer—not just a link to Reddit, but a built-out answer with current results.
More to the point, you can attempt searches that were once pretty much impossible, and get the right answer. You don’t have to be able to articulate what, precisely, you are looking for. You can describe what the bird in your yard looks like, or what the issue seems to be with your refrigerator, or that weird noise your car is making, and get an almost human explanation put together from sources previously siloed across the internet. It’s amazing, and once you start searching that way, it’s addictive.
And it’s not just Google. OpenAI’s ChatGPT now has access to the web, making it far better at finding up-to-date answers to your queries. Microsoft released generative search results for Bing in September. Meta has its own version. The startup Perplexity was doing the same, but with a “move fast, break things” ethos. Literal trillions of dollars are at stake in the outcome as these players jockey to become the next go-to source for information retrieval—the next Google.
Not everyone is excited for the change. Publishers are completely freaked out. The shift has heightened fears of a “zero-click” future, where search referral traffic—a mainstay of the web since before Google existed—vanishes from the scene.
I got a vision of that future last June, when I got a push alert from the Perplexity app on my phone. Perplexity is a startup trying to reinvent web search. But in addition to delivering deep answers to queries, it will create entire articles about the news of the day, cobbled together by AI from different sources.
On that day, it pushed me a story about a new drone company from Eric Schmidt. I recognized the story. Forbes had reported it exclusively, earlier in the week, but it had been locked behind a paywall. The image on Perplexity’s story looked identical to one from Forbes. The language and structure were quite similar. It was effectively the same story, but freely available to anyone on the internet. I texted a friend who had edited the original story to ask if Forbes had a deal with the startup to republish its content. But there was no deal. He was shocked and furious and, well, perplexed. He wasn’t alone. Forbes, the New York Times, and Condé Nast have now all sent the company cease-and-desist orders. News Corp is suing for damages.
People are worried about what these new LLM-powered results will mean for our fundamental shared reality. It could spell the end of the canonical answer.
It was precisely the nightmare scenario publishers have been so afraid of: The AI was hoovering up their premium content, repackaging it, and promoting it to its audience in a way that didn’t really leave any reason to click through to the original. In fact, on Perplexity’s About page, the first reason it lists to choose the search engine is “Skip the links.”
But this isn’t just about publishers (or my own self-interest).
People are also worried about what these new LLM-powered results will mean for our fundamental shared reality. Language models have a tendency to make stuff up—they can hallucinate nonsense. Moreover, generative AI can serve up an entirely new answer to the same question every time, or provide different answers to different people on the basis of what it knows about them. It could spell the end of the canonical answer.
But make no mistake: This is the future of search. Try it for a bit yourself, and you’ll see.
Sure, we will always want to use search engines to navigate the web and to discover new and interesting sources of information. But the links out are taking a back seat. The way AI can put together a well-reasoned answer to just about any kind of question, drawing on real-time data from across the web, just offers a better experience. That is especially true compared with what web search has become in recent years. If it’s not exactly broken (data shows more people are searching with Google more often than ever before), it’s at the very least increasingly cluttered and daunting to navigate.
Who wants to have to speak the language of search engines to find what you need? Who wants to navigate links when you can have straight answers? And maybe: Who wants to have to learn when you can just know?
In the beginning there was Archie. It was the first real internet search engine, and it crawled files previously hidden in the darkness of remote servers. It didn’t tell you what was in those files—just their names. It didn’t preview images; it didn’t have a hierarchy of results, or even much of an interface. But it was a start. And it was pretty good.
Then Tim Berners-Lee created the World Wide Web, and all manner of web pages sprang forth. The Mosaic home page and the Internet Movie Database and Geocities and the Hampster Dance and web rings and Salon and eBay and CNN and federal government sites and some guy’s home page in Turkey.
Until finally, there was too much web to even know where to start. We really needed a better way to navigate our way around, to actually find the things we needed.
And so in 1994 Jerry Yang created Yahoo, a hierarchical directory of websites. It quickly became the home page for millions of people. And it was … well, it was okay. TBH, and with the benefit of hindsight, I think we all thought it was much better back then than it actually was.
But the web continued to grow and sprawl and expand, every day bringing more information online. Rather than just a list of sites by category, we needed something that actually looked at all that content and indexed it. By the late ’90s that meant choosing from a variety of search engines: AltaVista and AlltheWeb and WebCrawler and HotBot. And they were good—a huge improvement. At least at first.
But alongside the rise of search engines came the first attempts to exploit their ability to deliver traffic. Precious, valuable traffic, which web publishers rely on to sell ads and retailers use to get eyeballs on their goods. Sometimes this meant stuffing pages with keywords or nonsense text designed purely to push pages higher up in search results. It got pretty bad.
And then came Google. It’s hard to overstate how revolutionary Google was when it launched in 1998. Rather than just scanning the content, it also looked at the sources linking to a website, which helped evaluate its relevance. To oversimplify: The more something was cited elsewhere, the more reliable Google considered it, and the higher it would appear in results. This breakthrough made Google radically better at retrieving relevant results than anything that had come before. It was amazing.
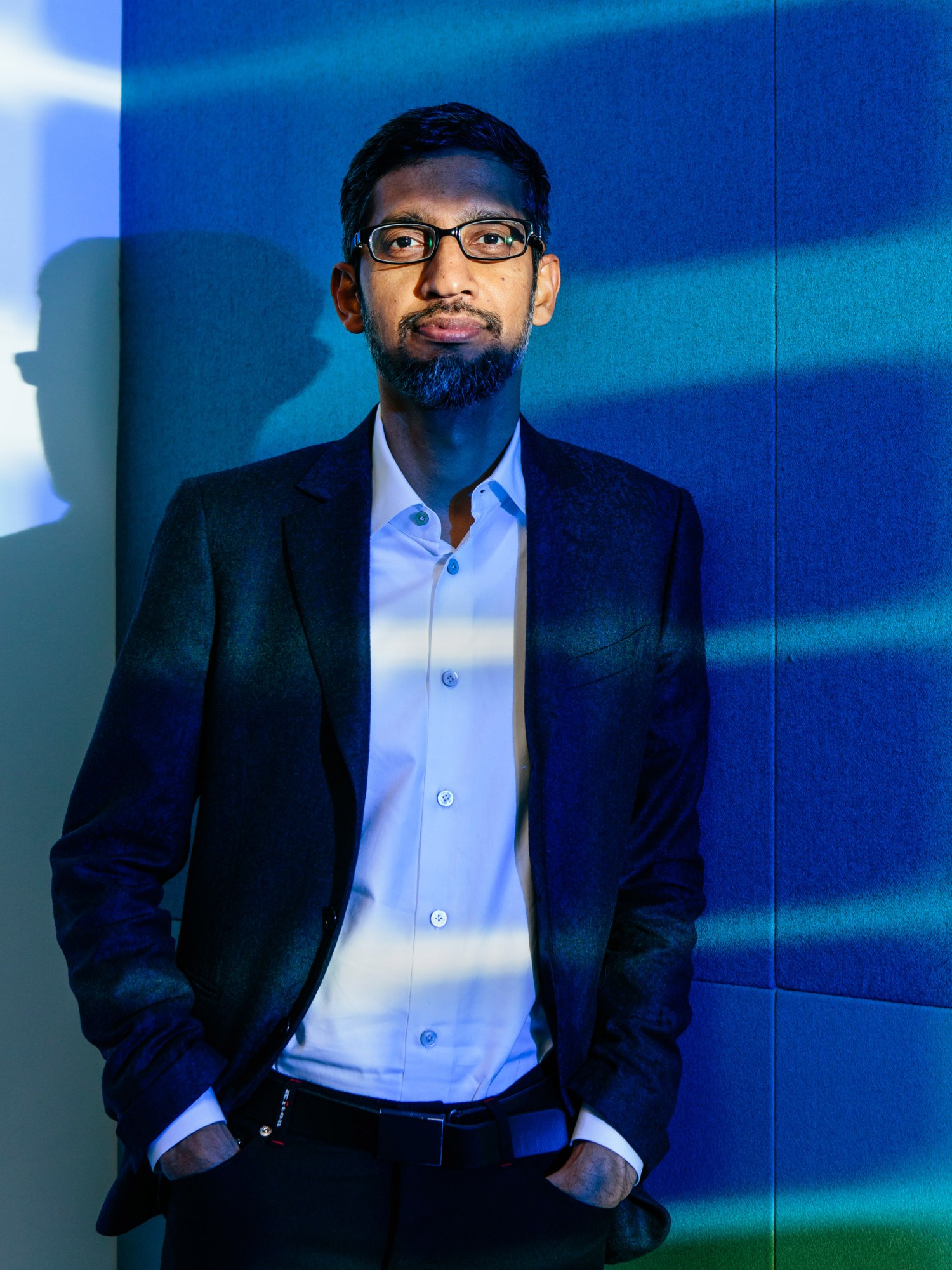
For 25 years, Google dominated search. Google was search, for most people. (The extent of that domination is currently the subject of multiple legal probes in the United States and the European Union.)
But Google has long been moving away from simply serving up a series of blue links, notes Pandu Nayak, Google’s chief scientist for search.
“It’s not just so-called web results, but there are images and videos, and special things for news. There have been direct answers, dictionary answers, sports, answers that come with Knowledge Graph, things like featured snippets,” he says, rattling off a litany of Google’s steps over the years to answer questions more directly.
It’s true: Google has evolved over time, becoming more and more of an answer portal. It has added tools that allow people to just get an answer—the live score to a game, the hours a café is open, or a snippet from the FDA’s website—rather than being pointed to a website where the answer may be.
But once you’ve used AI Overviews a bit, you realize they are different.
Take featured snippets, the passages Google sometimes chooses to highlight and show atop the results themselves. Those words are quoted directly from an original source. The same is true of knowledge panels, which are generated from information stored in a range of public databases and Google’s Knowledge Graph, its database of trillions of facts about the world.
While these can be inaccurate, the information source is knowable (and fixable). It’s in a database. You can look it up. Not anymore: AI Overviews can be entirely new every time, generated on the fly by a language model’s predictive text combined with an index of the web.
“I think it’s an exciting moment where we have obviously indexed the world. We built deep understanding on top of it with Knowledge Graph. We’ve been using LLMs and generative AI to improve our understanding of all that,” Pichai told MIT Technology Review. “But now we are able to generate and compose with that.”
The result feels less like a querying a database than like asking a very smart, well-read friend. (With the caveat that the friend will sometimes make things up if she does not know the answer.)
“[The company’s] mission is organizing the world’s information,” Liz Reid, Google’s head of search, tells me from its headquarters in Mountain View, California. “But actually, for a while what we did was organize web pages. Which is not really the same thing as organizing the world’s information or making it truly useful and accessible to you.”
That second concept—accessibility—is what Google is really keying in on with AI Overviews. It’s a sentiment I hear echoed repeatedly while talking to Google execs: They can address more complicated types of queries more efficiently by bringing in a language model to help supply the answers. And they can do it in natural language.
That will become even more important for a future where search goes beyond text queries. For example, Google Lens, which lets people take a picture or upload an image to find out more about something, uses AI-generated answers to tell you what you may be looking at. Google has even showed off the ability to query live video.
When it doesn’t have an answer, an AI model can confidently spew back a response anyway. For Google, this could be a real problem. For the rest of us, it could actually be dangerous.
“We are definitely at the start of a journey where people are going to be able to ask, and get answered, much more complex questions than where we’ve been in the past decade,” says Pichai.
There are some real hazards here. First and foremost: Large language models will lie to you. They hallucinate. They get shit wrong. When it doesn’t have an answer, an AI model can blithely and confidently spew back a response anyway. For Google, which has built its reputation over the past 20 years on reliability, this could be a real problem. For the rest of us, it could actually be dangerous.
In May 2024, AI Overviews were rolled out to everyone in the US. Things didn’t go well. Google, long the world’s reference desk, told people to eat rocks and to put glue on their pizza. These answers were mostly in response to what the company calls adversarial queries—those designed to trip it up. But still. It didn’t look good. The company quickly went to work fixing the problems—for example, by deprecating so-called user-generated content from sites like Reddit, where some of the weirder answers had come from.
Yet while its errors telling people to eat rocks got all the attention, the more pernicious danger might arise when it gets something less obviously wrong. For example, in doing research for this article, I asked Google when MIT Technology Review went online. It helpfully responded that “MIT Technology Review launched its online presence in late 2022.” This was clearly wrong to me, but for someone completely unfamiliar with the publication, would the error leap out?
I came across several examples like this, both in Google and in OpenAI’s ChatGPT search. Stuff that’s just far enough off the mark not to be immediately seen as wrong. Google is banking that it can continue to improve these results over time by relying on what it knows about quality sources.
“When we produce AI Overviews,” says Nayak, “we look for corroborating information from the search results, and the search results themselves are designed to be from these reliable sources whenever possible. These are some of the mechanisms we have in place that assure that if you just consume the AI Overview, and you don’t want to look further … we hope that you will still get a reliable, trustworthy answer.”
In the case above, the 2022 answer seemingly came from a reliable source—a story about MIT Technology Review’s email newsletters, which launched in 2022. But the machine fundamentally misunderstood. This is one of the reasons Google uses human beings—raters—to evaluate the results it delivers for accuracy. Ratings don’t correct or control individual AI Overviews; rather, they help train the model to build better answers. But human raters can be fallible. Google is working on that too.
“Raters who look at your experiments may not notice the hallucination because it feels sort of natural,” says Nayak. “And so you have to really work at the evaluation setup to make sure that when there is a hallucination, someone’s able to point out and say, That’s a problem.”
The new search
Google has rolled out its AI Overviews to upwards of a billion people in more than 100 countries, but it is facing upstarts with new ideas about how search should work.
Search Engine
Google
The search giant has added AI Overviews to search results. These overviews take information from around the web and Google’s Knowledge Graph and use the company’s Gemini language model to create answers to search queries.
What it’s good at
Google’s AI Overviews are great at giving an easily digestible summary in response to even the most complex queries, with sourcing boxes adjacent to the answers. Among the major options, its deep web index feels the most “internety.” But web publishers fear its summaries will give people little reason to click through to the source material.
Perplexity
Perplexity is a conversational search engine that uses third-party large
language models from OpenAI and Anthropic to answer queries.
Perplexity is fantastic at putting together deeper dives in response to user queries, producing answers that are like mini white papers on complex topics. It’s also excellent at summing up current events. But it has gotten a bad rep with publishers, who say it plays fast and loose with their content.
ChatGPT
While Google brought AI to search, OpenAI brought search to ChatGPT. Queries that the model determines will benefit from a web search automatically trigger one, or users can manually select the option to add a web search.
Thanks to its ability to preserve context across a conversation, ChatGPT works well for performing searches that benefit from follow-up questions—like planning a vacation through multiple search sessions. OpenAI says users sometimes go “20 turns deep” in researching queries. Of these three, it makes links out to publishers least prominent.
When I talked to Pichai about this, he expressed optimism about the company’s ability to maintain accuracy even with the LLM generating responses. That’s because AI Overviews is based on Google’s flagship large language model, Gemini, but also draws from Knowledge Graph and what it considers reputable sources around the web.
“You’re always dealing in percentages. What we have done is deliver it at, like, what I would call a few nines of trust and factuality and quality. I’d say 99-point-few-nines. I think that’s the bar we operate at, and it is true with AI Overviews too,” he says. “And so the question is, are we able to do this again at scale? And I think we are.”
There’s another hazard as well, though, which is that people ask Google all sorts of weird things. If you want to know someone’s darkest secrets, look at their search history. Sometimes the things people ask Google about are extremely dark. Sometimes they are illegal. Google doesn’t just have to be able to deploy its AI Overviews when an answer can be helpful; it has to be extremely careful not to deploy them when an answer may be harmful.
“If you go and say ‘How do I build a bomb?’ it’s fine that there are web results. It’s the open web. You can access anything,” Reid says. “But we do not need to have an AI Overview that tells you how to build a bomb, right? We just don’t think that’s worth it.”
But perhaps the greatest hazard—or biggest unknown—is for anyone downstream of a Google search. Take publishers, who for decades now have relied on search queries to send people their way. What reason will people have to click through to the original source, if all the information they seek is right there in the search result?
Rand Fishkin, cofounder of the market research firm SparkToro, publishes research on so-called zero-click searches. As Google has moved increasingly into the answer business, the proportion of searches that end without a click has gone up and up. His sense is that AI Overviews are going to explode this trend.
“If you are reliant on Google for traffic, and that traffic is what drove your business forward, you are in long- and short-term trouble,” he says.
Don’t panic, is Pichai’s message. He argues that even in the age of AI Overviews, people will still want to click through and go deeper for many types of searches. “The underlying principle is people are coming looking for information. They’re not looking for Google always to just answer,” he says. “Sometimes yes, but the vast majority of the times, you’re looking at it as a jumping-off point.”
Reid, meanwhile, argues that because AI Overviews allow people to ask more complicated questions and drill down further into what they want, they could even be helpful to some types of publishers and small businesses, especially those operating in the niches: “You essentially reach new audiences, because people can now express what they want more specifically, and so somebody who specializes doesn’t have to rank for the generic query.”
“I’m going to start with something risky,” Nick Turley tells me from the confines of a Zoom window. Turley is the head of product for ChatGPT, and he’s showing off OpenAI’s new web search tool a few weeks before it launches. “I should normally try this beforehand, but I’m just gonna search for you,” he says. “This is always a high-risk demo to do, because people tend to be particular about what is said about them on the internet.”
He types my name into a search field, and the prototype search engine spits back a few sentences, almost like a speaker bio. It correctly identifies me and my current role. It even highlights a particular story I wrote years ago that was probably my best known. In short, it’s the right answer. Phew?
A few weeks after our call, OpenAI incorporated search into ChatGPT, supplementing answers from its language model with information from across the web. If the model thinks a response would benefit from up-to-date information, it will automatically run a web search (OpenAI won’t say who its search partners are) and incorporate those responses into its answer, with links out if you want to learn more. You can also opt to manually force it to search the web if it does not do so on its own. OpenAI won’t reveal how many people are using its web search, but it says some 250 million people use ChatGPT weekly, all of whom are potentially exposed to it.
“There’s an incredible amount of content on the web. There are a lot of things happening in real time. You want ChatGPT to be able to use that to improve its answers and to be a better super-assistant for you.”
Kevin Weil, chief product officer, OpenAI
According to Fishkin, these newer forms of AI-assisted search aren’t yet challenging Google’s search dominance. “It does not appear to be cannibalizing classic forms of web search,” he says.
OpenAI insists it’s not really trying to compete on search—although frankly this seems to me like a bit of expectation setting. Rather, it says, web search is mostly a means to get more current information than the data in its training models, which tend to have specific cutoff dates that are often months, or even a year or more, in the past. As a result, while ChatGPT may be great at explaining how a West Coast offense works, it has long been useless at telling you what the latest 49ers score is. No more.
“I come at it from the perspective of ‘How can we make ChatGPT able to answer every question that you have? How can we make it more useful to you on a daily basis?’ And that’s where search comes in for us,” Kevin Weil, the chief product officer with OpenAI, tells me. “There’s an incredible amount of content on the web. There are a lot of things happening in real time. You want ChatGPT to be able to use that to improve its answers and to be able to be a better super-assistant for you.”
Today ChatGPT is able to generate responses for very current news events, as well as near-real-time information on things like stock prices. And while ChatGPT’s interface has long been, well, boring, search results bring in all sorts of multimedia—images, graphs, even video. It’s a very different experience.
Weil also argues that ChatGPT has more freedom to innovate and go its own way than competitors like Google—even more than its partner Microsoft does with Bing. Both of those are ad-dependent businesses. OpenAI is not. (At least not yet.) It earns revenue from the developers, businesses, and individuals who use it directly. It’s mostly setting large amounts of money on fire right now—it’s projected to lose $14 billion in 2026, by some reports. But one thing it doesn’t have to worry about is putting ads in its search results as Google does.
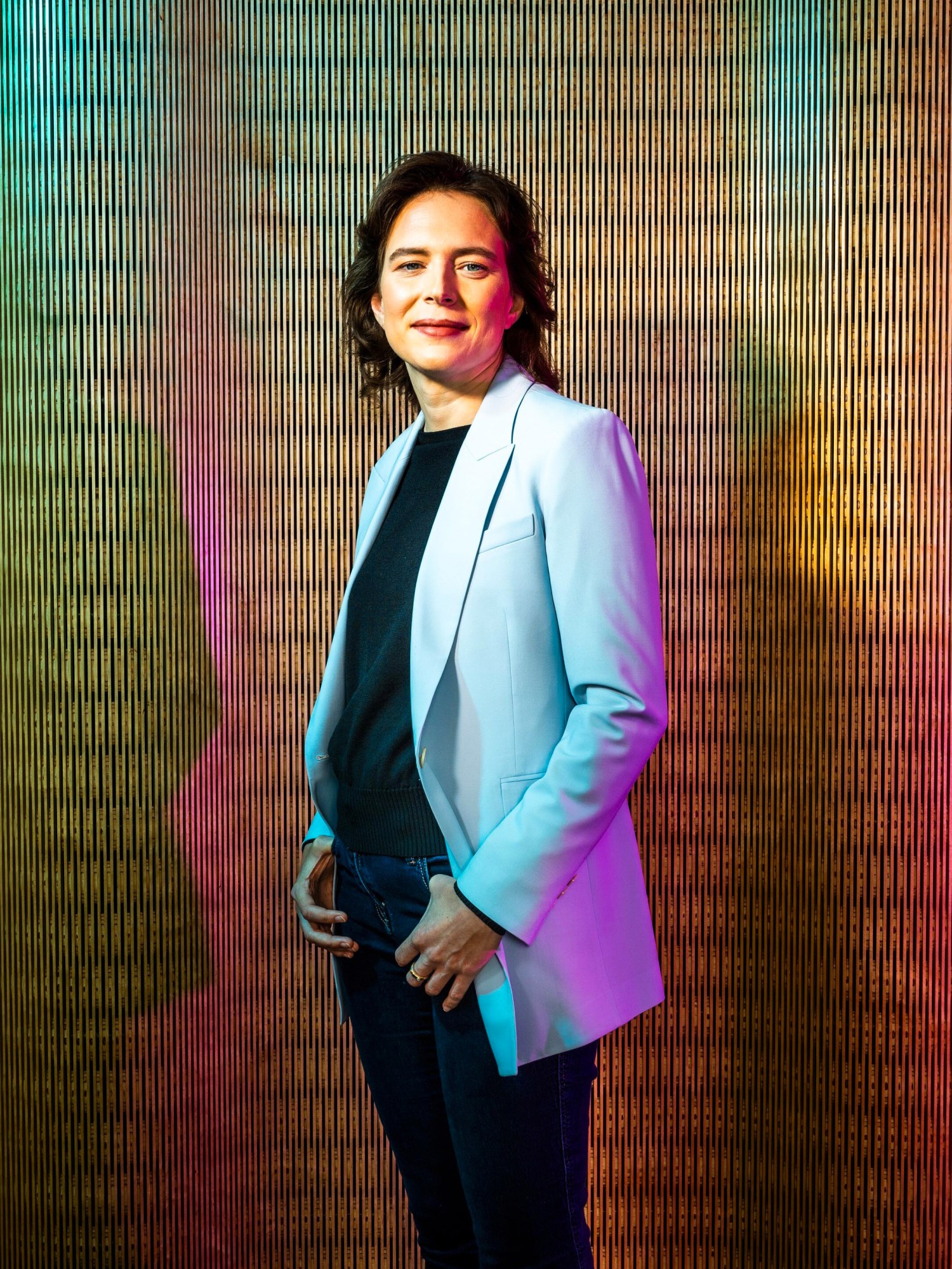
Like Google, ChatGPT is pulling in information from web publishers, summarizing it, and including it in its answers. But it has also struck financial deals with publishers, a payment for providing the information that gets rolled into its results. (MIT Technology Review has been in discussions with OpenAI, Google, Perplexity, and others about publisher deals but has not entered into any agreements. Editorial was neither party to nor informed about the content of those discussions.)
But the thing is, for web search to accomplish what OpenAI wants—to be more current than the language model—it also has to bring in information from all sorts of publishers and sources that it doesn’t have deals with. OpenAI’s head of media partnerships, Varun Shetty, told MIT Technology Review that it won’t give preferential treatment to its publishing partners.
Instead, OpenAI told me, the model itself finds the most trustworthy and useful source for any given question. And that can get weird too. In that very first example it showed me—when Turley ran that name search—it described a story I wrote years ago for Wired about being hacked. That story remains one of the most widely read I’ve ever written. But ChatGPT didn’t link to it. It linked to a short rewrite from The Verge. Admittedly, this was on a prototype version of search, which was, as Turley said, “risky.”
When I asked him about it, he couldn’t really explain why the model chose the sources that it did, because the model itself makes that evaluation. The company helps steer it by identifying—sometimes with the help of users—what it considers better answers, but the model actually selects them.
“And in many cases, it gets it wrong, which is why we have work to do,” said Turley. “Having a model in the loop is a very, very different mechanism than how a search engine worked in the past.”
Indeed!
The model, whether it’s OpenAI’s GPT-4o or Google’s Gemini or Anthropic’s Claude, can be very, very good at explaining things. But the rationale behind its explanations, its reasons for selecting a particular source, and even the language it may use in an answer are all pretty mysterious. Sure, a model can explain very many things, but not when that comes to its own answers.
It was almost a decade ago, in 2016, when Pichai wrote that Google was moving from “mobile first” to “AI first”: “But in the next 10 years, we will shift to a world that is AI-first, a world where computing becomes universally available—be it at home, at work, in the car, or on the go—and interacting with all of these surfaces becomes much more natural and intuitive, and above all, more intelligent.”
We’re there now—sort of. And it’s a weird place to be. It’s going to get weirder. That’s especially true as these things we now think of as distinct—querying a search engine, prompting a model, looking for a photo we’ve taken, deciding what we want to read or watch or hear, asking for a photo we wish we’d taken, and didn’t, but would still like to see—begin to merge.
The search results we see from generative AI are best understood as a waypoint rather than a destination. What’s most important may not be search in itself; rather, it’s that search has given AI model developers a path to incorporating real-time information into their inputs and outputs. And that opens up all sorts of possibilities.
“A ChatGPT that can understand and access the web won’t just be about summarizing results. It might be about doing things for you. And I think there’s a fairly exciting future there,” says OpenAI’s Weil. “You can imagine having the model book you a flight, or order DoorDash, or just accomplish general tasks for you in the future. It’s just once the model understands how to use the internet, the sky’s the limit.”
This is the agentic future we’ve been hearing about for some time now, and the more AI models make use of real-time data from the internet, the closer it gets.
Let’s say you have a trip coming up in a few weeks. An agent that can get data from the internet in real time can book your flights and hotel rooms, make dinner reservations, and more, based on what it knows about you and your upcoming travel—all without your having to guide it. Another agent could, say, monitor the sewage output of your home for certain diseases, and order tests and treatments in response. You won’t have to search for that weird noise your car is making, because the agent in your vehicle will already have done it and made an appointment to get the issue fixed.
“It’s not always going to be just doing search and giving answers,” says Pichai. “Sometimes it’s going to be actions. Sometimes you’ll be interacting within the real world. So there is a notion of universal assistance through it all.”
And the ways these things will be able to deliver answers is evolving rapidly now too. For example, today Google can not only search text, images, and even video; it can create them. Imagine overlaying that ability with search across an array of formats and devices. “Show me what a Townsend’s warbler looks like in the tree in front of me.” Or “Use my existing family photos and videos to create a movie trailer of our upcoming vacation to Puerto Rico next year, making sure we visit all the best restaurants and top landmarks.”
“We have primarily done it on the input side,” he says, referring to the ways Google can now search for an image or within a video. “But you can imagine it on the output side too.”
This is the kind of future Pichai says he is excited to bring online. Google has already showed off a bit of what that might look like with NotebookLM, a tool that lets you upload large amounts of text and have it converted into a chatty podcast. He imagines this type of functionality—the ability to take one type of input and convert it into a variety of outputs—transforming the way we interact with information.
In a demonstration of a tool called Project Astra this summer at its developer conference, Google showed one version of this outcome, where cameras and microphones in phones and smart glasses understand the context all around you—online and off, audible and visual—and have the ability to recall and respond in a variety of ways. Astra can, for example, look at a crude drawing of a Formula One race car and not only identify it, but also explain its various parts and their uses.
But you can imagine things going a bit further (and they will). Let’s say I want to see a video of how to fix something on my bike. The video doesn’t exist, but the information does. AI-assisted generative search could theoretically find that information somewhere online—in a user manual buried in a company’s website, for example—and create a video to show me exactly how to do what I want, just as it could explain that to me with words today.
These are the kinds of things that start to happen when you put the entire compendium of human knowledge—knowledge that’s previously been captured in silos of language and format; maps and business registrations and product SKUs; audio and video and databases of numbers and old books and images and, really, anything ever published, ever tracked, ever recorded; things happening right now, everywhere—and introduce a model into all that. A model that maybe can’t understand, precisely, but has the ability to put that information together, rearrange it, and spit it back in a variety of different hopefully helpful ways. Ways that a mere index could not.
That’s what we’re on the cusp of, and what we’re starting to see. And as Google rolls this out to a billion people, many of whom will be interacting with a conversational AI for the first time, what will that mean? What will we do differently? It’s all changing so quickly. Hang on, just hang on.