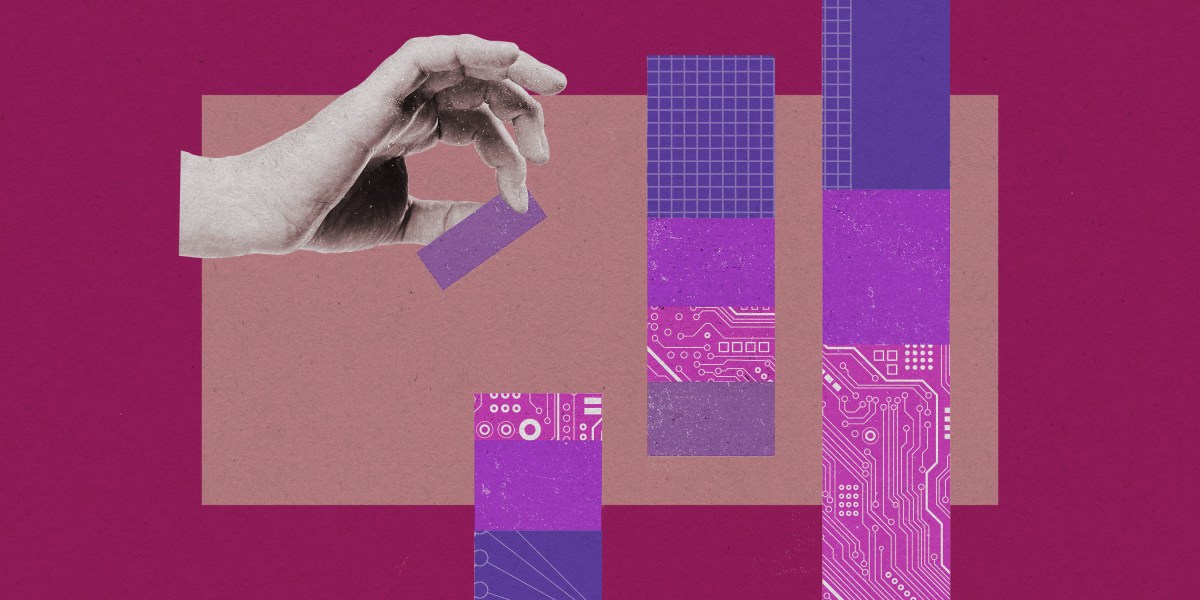
It’s not easy being one of Silicon Valley’s favorite benchmarks.
SWE-Bench (pronounced “swee bench”) launched in November 2024 to evaluate an AI model’s coding skill, using more than 2,000 real-world programming problems pulled from the public GitHub repositories of 12 different Python-based projects.
In the months since then, it’s quickly become one of the most popular tests in AI. A SWE-Bench score has become a mainstay of major model releases from OpenAI, Anthropic, and Google—and outside of foundation models, the fine-tuners at AI firms are in constant competition to see who can rise above the pack. The top of the leaderboard is a pileup between three different fine tunings of Anthropic’s Claude Sonnet model and Amazon’s Q developer agent. Auto Code Rover—one of the Claude modifications—nabbed the number two spot in November, and was acquired just three months later.
Despite all the fervor, this isn’t exactly a truthful assessment of which model is “better.” As the benchmark has gained prominence, “you start to see that people really want that top spot,” says John Yang, a researcher on the team that developed SWE-Bench at Princeton University. As a result, entrants have begun to game the system—which is pushing many others to wonder whether there’s a better way to actually measure AI achievement.
Developers of these coding agents aren’t necessarily doing anything as straightforward cheating, but they’re crafting approaches that are too neatly tailored to the specifics of the benchmark. The initial SWE-Bench test set was limited to programs written in Python, which meant developers could gain an advantage by training their models exclusively on Python code. Soon, Yang noticed that high-scoring models would fail completely when tested on different programming languages—revealing an approach to the test that he describes as “gilded.”
“It looks nice and shiny at first glance, but then you try to run it on a different language and the whole thing just kind of falls apart,” Yang says. “At that point, you’re not designing a software engineering agent. You’re designing to make a SWE-Bench agent, which is much less interesting.”
The SWE-Bench issue is a symptom of a more sweeping—and complicated—problem in AI evaluation, and one that’s increasingly sparking heated debate: The benchmarks the industry uses to guide development are drifting further and further away from evaluating actual capabilities, calling their basic value into question. Making the situation worse, several benchmarks, most notably FrontierMath and Chatbot Arena, have recently come under heat for an alleged lack of transparency. Nevertheless, benchmarks still play a central role in model development, even if few experts are willing to take their results at face value. OpenAI cofounder Andrej Karpathy recently described the situation as “an evaluation crisis”: the industry has fewer trusted methods for measuring capabilities and no clear path to better ones.
“Historically, benchmarks were the way we evaluated AI systems,” says Vanessa Parli, director of research at Stanford University’s Institute for Human-Centered AI. “Is that the way we want to evaluate systems going forward? And if it’s not, what is the way?”
A growing group of academics and AI researchers are making the case that the answer is to go smaller, trading sweeping ambition for an approach inspired by the social sciences. Specifically, they want to focus more on testing validity, which for quantitative social scientists refers to how well a given questionnaire measures what it’s claiming to measure—and, more fundamentally, whether what it is measuring has a coherent definition. That could cause trouble for benchmarks assessing hazily defined concepts like “reasoning” or “scientific knowledge”—and for developers aiming to reach the much–hyped goal of artificial general intelligence—but it would put the industry on firmer ground as it looks to prove the worth of individual models.
“Taking validity seriously means asking folks in academia, industry, or wherever to show that their system does what they say it does,” says Abigail Jacobs, a University of Michigan professor who is a central figure in the new push for validity. “I think it points to a weakness in the AI world if they want to back off from showing that they can support their claim.”
The limits of traditional testing
If AI companies have been slow to respond to the growing failure of benchmarks, it’s partially because the test-scoring approach has been so effective for so long.
One of the biggest early successes of contemporary AI was the ImageNet challenge, a kind of antecedent to contemporary benchmarks. Released in 2010 as an open challenge to researchers, the database held more than 3 million images for AI systems to categorize into 1,000 different classes.
Crucially, the test was completely agnostic to methods, and any successful algorithm quickly gained credibility regardless of how it worked. When an algorithm called AlexNet broke through in 2012, with a then unconventional form of GPU training, it became one of the foundational results of modern AI. Few would have guessed in advance that AlexNet’s convolutional neural nets would be the secret to unlocking image recognition—but after it scored well, no one dared dispute it. (One of AlexNet’s developers, Ilya Sutskever, would go on to cofound OpenAI.)
A large part of what made this challenge so effective was that there was little practical difference between ImageNet’s object classification challenge and the actual process of asking a computer to recognize an image. Even if there were disputes about methods, no one doubted that the highest-scoring model would have an advantage when deployed in an actual image recognition system.
But in the 12 years since, AI researchers have applied that same method-agnostic approach to increasingly general tasks. SWE-Bench is commonly used as a proxy for broader coding ability, while other exam-style benchmarks often stand in for reasoning ability. That broad scope makes it difficult to be rigorous about what a specific benchmark measures—which, in turn, makes it hard to use the findings responsibly.
Where things break down
Anka Reuel, a PhD student who has been focusing on the benchmark problem as part of her research at Stanford, has become convinced the evaluation problem is the result of this push toward generality. “We’ve moved from task-specific models to general-purpose models,” Reuel says. “It’s not about a single task anymore but a whole bunch of tasks, so evaluation becomes harder.”
Like the University of Michigan’s Jacobs, Reuel thinks “the main issue with benchmarks is validity, even more than the practical implementation,” noting: “That’s where a lot of things break down.” For a task as complicated as coding, for instance, it’s nearly impossible to incorporate every possible scenario into your problem set. As a result, it’s hard to gauge whether a model is scoring better because it’s more skilled at coding or because it has more effectively manipulated the problem set. And with so much pressure on developers to achieve record scores, shortcuts are hard to resist.
For developers, the hope is that success on lots of specific benchmarks will add up to a generally capable model. But the techniques of agentic AI mean a single AI system can encompass a complex array of different models, making it hard to evaluate whether improvement on a specific task will lead to generalization. “There’s just many more knobs you can turn,” says Sayash Kapoor, a computer scientist at Princeton and a prominent critic of sloppy practices in the AI industry. “When it comes to agents, they have sort of given up on the best practices for evaluation.”
In a paper from last July, Kapoor called out specific issues in how AI models were approaching the WebArena benchmark, designed by Carnegie Mellon University researchers in 2024 as a test of an AI agent’s ability to traverse the web. The benchmark consists of more than 800 tasks to be performed on a set of cloned websites mimicking Reddit, Wikipedia, and others. Kapoor and his team identified an apparent hack in the winning model, called STeP. STeP included specific instructions about how Reddit structures URLs, allowing STeP models to jump directly to a given user’s profile page (a frequent element of WebArena tasks).
This shortcut wasn’t exactly cheating, but Kapoor sees it as “a serious misrepresentation of how well the agent would work had it seen the tasks in WebArena for the first time.” Because the technique was successful, though, a similar policy has since been adopted by OpenAI’s web agent Operator. (“Our evaluation setting is designed to assess how well an agent can solve tasks given some instruction about website structures and task execution,” an OpenAI representative said when reached for comment. “This approach is consistent with how others have used and reported results with WebArena.” STeP did not respond to a request for comment.)
Further highlighting the problem with AI benchmarks, late last month Kapoor and a team of researchers wrote a paper that revealed significant problems in Chatbot Arena, the popular crowdsourced evaluation system. According to the paper, the leaderboard was being manipulated; many top foundation models were conducting undisclosed private testing and releasing their scores selectively.
Today, even ImageNet itself, the mother of all benchmarks, has started to fall victim to validity problems. A 2023 study from researchers at the University of Washington and Google Research found that when ImageNet-winning algorithms were pitted against six real-world data sets, the architecture improvement “resulted in little to no progress,” suggesting that the external validity of the test had reached its limit.
Going smaller
For those who believe the main problem is validity, the best fix is reconnecting benchmarks to specific tasks. As Reuel puts it, AI developers “have to resort to these high-level benchmarks that are almost meaningless for downstream consumers, because the benchmark developers can’t anticipate the downstream task anymore.” So what if there was a way to help the downstream consumers identify this gap?
In November 2024, Reuel launched a public ranking project called BetterBench, which rates benchmarks on dozens of different criteria, such as whether the code has been publicly documented. But validity is a central theme, with particular criteria challenging designers to spell out what capability their benchmark is testing and how it relates to the tasks that make up the benchmark.
“You need to have a structural breakdown of the capabilities,” Reuel says. “What are the actual skills you care about, and how do you operationalize them into something we can measure?”
The results are surprising. One of the highest-scoring benchmarks is also the oldest: the Arcade Learning Environment (ALE), established in 2013 as a way to test models’ ability to learn how to play a library of Atari 2600 games. One of the lowest-scoring is the Massive Multitask Language Understanding (MMLU) benchmark, a widely used test for general language skills; by the standards of BetterBench, the connection between the questions and the underlying skill was too poorly defined.
BetterBench hasn’t meant much for the reputations of specific benchmarks, at least not yet; MMLU is still widely used, and ALE is still marginal. But the project has succeeded in pushing validity into the broader conversation about how to fix benchmarks. In April, Reuel quietly joined a new research group hosted by Hugging Face, the University of Edinburgh, and EleutherAI, where she’ll develop her ideas on validity and AI model evaluation with other figures in the field. (An official announcement is expected later this month.)
Irene Solaiman, Hugging Face’s head of global policy, says the group will focus on building valid benchmarks that go beyond measuring straightforward capabilities. “There’s just so much hunger for a good benchmark off the shelf that already works,” Solaiman says. “A lot of evaluations are trying to do too much.”
Increasingly, the rest of the industry seems to agree. In a paper in March, researchers from Google, Microsoft, Anthropic, and others laid out a new framework for improving evaluations—with validity as the first step.
“AI evaluation science must,” the researchers argue, “move beyond coarse grained claims of ‘general intelligence’ towards more task-specific and real-world relevant measures of progress.”
Measuring the “squishy” things
To help make this shift, some researchers are looking to the tools of social science. A February position paper argued that “evaluating GenAI systems is a social science measurement challenge,” specifically unpacking how the validity systems used in social measurements can be applied to AI benchmarking.
The authors, largely employed by Microsoft’s research branch but joined by academics from Stanford and the University of Michigan, point to the standards that social scientists use to measure contested concepts like ideology, democracy, and media bias. Applied to AI benchmarks, those same procedures could offer a way to measure concepts like “reasoning” and “math proficiency” without slipping into hazy generalizations.
In the social science literature, it’s particularly important that metrics begin with a rigorous definition of the concept measured by the test. For instance, if the test is to measure how democratic a society is, it first needs to establish a definition for a “democratic society” and then establish questions that are relevant to that definition.
To apply this to a benchmark like SWE-Bench, designers would need to set aside the classic machine learning approach, which is to collect programming problems from GitHub and create a scheme to validate answers as true or false. Instead, they’d first need to define what the benchmark aims to measure (“ability to resolve flagged issues in software,” for instance), break that into subskills (different types of problems or types of program that the AI model can successfully process), and then finally assemble questions that accurately cover the different subskills.
It’s a profound change from how AI researchers typically approach benchmarking—but for researchers like Jacobs, a coauthor on the February paper, that’s the whole point. “There’s a mismatch between what’s happening in the tech industry and these tools from social science,” she says. “We have decades and decades of thinking about how we want to measure these squishy things about humans.”
Even though the idea has made a real impact in the research world, it’s been slow to influence the way AI companies are actually using benchmarks.
The last two months have seen new model releases from OpenAI, Anthropic, Google, and Meta, and all of them lean heavily on multiple-choice knowledge benchmarks like MMLU—the exact approach that validity researchers are trying to move past. After all, model releases are, for the most part, still about showing increases in general intelligence, and broad benchmarks continue to be used to back up those claims.
For some observers, that’s good enough. Benchmarks, Wharton professor Ethan Mollick says, are “bad measures of things, but also they’re what we’ve got.” He adds: “At the same time, the models are getting better. A lot of sins are forgiven by fast progress.”
For now, the industry’s long-standing focus on artificial general intelligence seems to be crowding out a more focused validity-based approach. As long as AI models can keep growing in general intelligence, then specific applications don’t seem as compelling—even if that leaves practitioners relying on tools they no longer fully trust.
“This is the tightrope we’re walking,” says Hugging Face’s Solaiman. “It’s too easy to throw the system out, but evaluations are really helpful in understanding our models, even with these limitations.”
Russell Brandom is a freelance writer covering artificial intelligence. He lives in Brooklyn with his wife and two cats.
This story was supported by a grant from the Tarbell Center for AI Journalism.