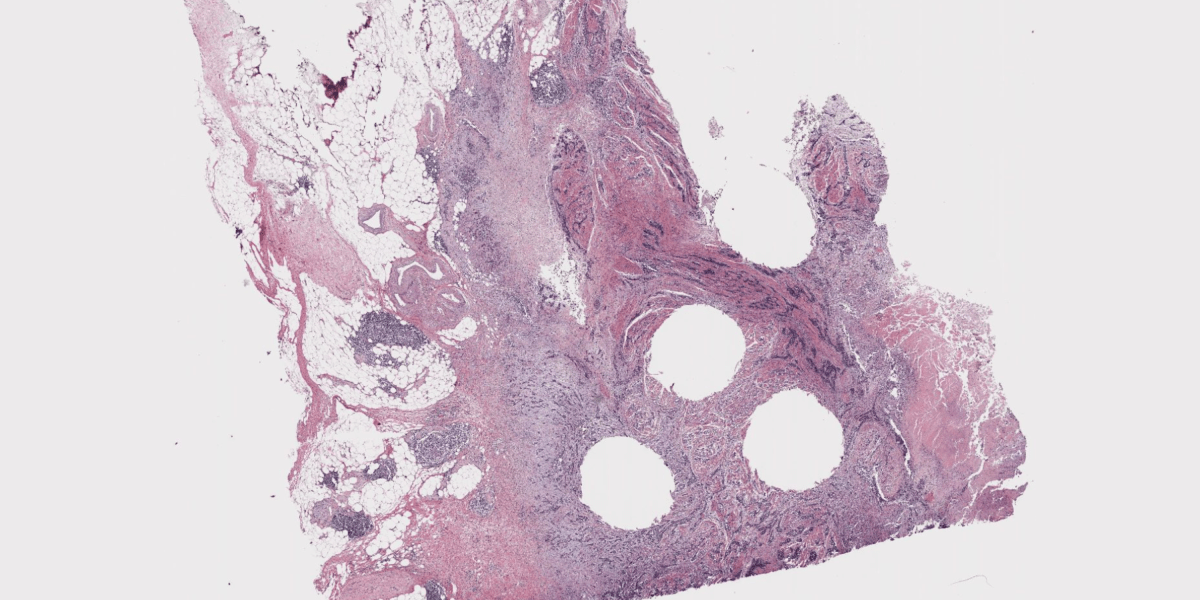
This story originally appeared in The Algorithm, our weekly newsletter on AI. To get stories like this in your inbox first, sign up here.
Peering into the body to find and diagnose cancer is all about spotting patterns. Radiologists use x-rays and magnetic resonance imaging to illuminate tumors, and pathologists examine tissue from kidneys, livers, and other areas under microscopes and look for patterns that show how severe a cancer is, whether particular treatments could work, and where the malignancy may spread.
In theory, artificial intelligence should be great at helping out. “Our job is pattern recognition,” says Andrew Norgan, a pathologist and medical director of the Mayo Clinic’s digital pathology platform. “We look at the slide and we gather pieces of information that have been proven to be important.”
Visual analysis is something that AI has gotten quite good at since the first image recognition models began taking off nearly 15 years ago. Even though no model will be perfect, you can imagine a powerful algorithm someday catching something that a human pathologist missed, or at least speeding up the process of getting a diagnosis. We’re starting to see lots of new efforts to build such a model—at least seven attempts in the last year alone—but they all remain experimental. What will it take to make them good enough to be used in the real world?
Details about the latest effort to build such a model, led by the AI health company Aignostics with the Mayo Clinic, were published on arXiv earlier this month. The paper has not been peer-reviewed, but it reveals much about the challenges of bringing such a tool to real clinical settings.
The model, called Atlas, was trained on 1.2 million tissue samples from 490,000 cases. Its accuracy was tested against six other leading AI pathology models. These models compete on shared tests like classifying breast cancer images or grading tumors, where the model’s predictions are compared with the correct answers given by human pathologists. Atlas beat rival models on six out of nine tests. It earned its highest score for categorizing cancerous colorectal tissue, reaching the same conclusion as human pathologists 97.1% of the time. For another task, though—classifying tumors from prostate cancer biopsies—Atlas beat the other models’ high scores with a score of just 70.5%. Its average across nine benchmarks showed that it got the same answers as human experts 84.6% of the time.
Let’s think about what this means. The best way to know what’s happening to cancerous cells in tissues is to have a sample examined by a pathologist, so that’s the performance that AI models are measured against. The best models are approaching humans in particular detection tasks but lagging behind in many others. So how good does a model have to be to be clinically useful?
“Ninety percent is probably not good enough. You need to be even better,” says Carlo Bifulco, chief medical officer at Providence Genomics and co-creator of GigaPath, one of the other AI pathology models examined in the Mayo Clinic study. But, Bifulco says, AI models that don’t score perfectly can still be useful in the short term, and could potentially help pathologists speed up their work and make diagnoses more quickly.
What obstacles are getting in the way of better performance? Problem number one is training data.
“Fewer than 10% of pathology practices in the US are digitized,” Norgan says. That means tissue samples are placed on slides and analyzed under microscopes, and then stored in massive registries without ever being documented digitally. Though European practices tend to be more digitized, and there are efforts underway to create shared data sets of tissue samples for AI models to train on, there’s still not a ton to work with.
Without diverse data sets, AI models struggle to identify the wide range of abnormalities that human pathologists have learned to interpret. That includes for rare diseases, says Maximilian Alber, cofounder and CTO of Aignostics. Scouring the publicly available databases for tissue samples of particularly rare diseases, “you’ll find 20 samples over 10 years,” he says.
Around 2022, the Mayo Clinic foresaw that this lack of training data would be a problem. It decided to digitize all of its own pathology practices moving forward, along with 12 million slides from its archives dating back decades (patients had consented to their being used for research). It hired a company to build a robot that began taking high-resolution photos of the tissues, working through up to a million samples per month. From these efforts, the team was able to collect the 1.2 million high-quality samples used to train the Mayo model.
This brings us to problem number two for using AI to spot cancer. Tissue samples from biopsies are tiny—often just a couple of millimeters in diameter—but are magnified to such a degree that digital images of them contain more than 14 billion pixels. That makes them about 287,000 times larger than images used to train the best AI image recognition models to date.
“That obviously means lots of storage costs and so forth,” says Hoifung Poon, an AI researcher at Microsoft who worked with Bifulco to create GigaPath, which was featured in Nature last year. But it also forces important decisions about which bits of the image you use to train the AI model, and which cells you might miss in the process. To make Atlas, the Mayo Clinic used what’s referred to as a tile method, essentially creating lots of snapshots from the same sample to feed into the AI model. Figuring out how to select these tiles is both art and science, and it’s not yet clear which ways of doing it lead to the best results.
Thirdly, there’s the question of which benchmarks are most important for a cancer-spotting AI model to perform well on. The Atlas researchers tested their model in the challenging domain of molecular-related benchmarks, which involves trying to find clues from sample tissue images to guess what’s happening on a molecular level. Here’s an example: Your body’s mismatch repair genes are of particular concern for cancer, because they catch errors made when your DNA gets replicated. If these errors aren’t caught, they can drive the development and progression of cancer.
“Some pathologists might tell you they kind of get a feeling when they think something’s mismatch-repair deficient based on how it looks,” Norgan says. But pathologists don’t act on that gut feeling alone. They can do molecular testing for a more definitive answer. What if instead, Norgan says, we can use AI to predict what’s happening on the molecular level? It’s an experiment: Could the AI model spot underlying molecular changes that humans can’t see?
Generally no, it turns out. Or at least not yet. Atlas’s average for the molecular testing was 44.9%. That’s the best performance for AI so far, but it shows this type of testing has a long way to go.
Bifulco says Atlas represents incremental but real progress. “My feeling, unfortunately, is that everybody’s stuck at a similar level,” he says. “We need something different in terms of models to really make dramatic progress, and we need larger data sets.”
Deeper Learning
OpenAI has created an AI model for longevity science
AI has long had its fingerprints on the science of protein folding. But OpenAI now says it’s created a model that can engineer proteins, turning regular cells into stem cells. That goal has been pursued by companies in longevity science, because stem cells can produce any other tissue in the body and, in theory, could be a starting point for rejuvenating animals, building human organs, or providing supplies of replacement cells.
Why it matters: The work was a product of OpenAI’s collaboration with the longevity company Retro Labs, in which Sam Altman invested $180 million. It represents OpenAI’s first model focused on biological data and its first public claim that its models can deliver scientific results. The AI model reportedly engineered more effective proteins, and more quickly, than the company’s scientists could. But outside scientists can’t evaluate the claims until the studies have been published. Read more from Antonio Regalado.
Bits and Bytes
What we know about the TikTok ban
The popular video app went dark in the United States late Saturday and then came back around noon on Sunday, even as a law banning it took effect. (The New York Times)
Why Meta might not end up like X
X lost lots of advertising dollars as Elon Musk changed the platform’s policies. But Facebook and Instagram’s massive scale make them hard platforms for advertisers to avoid. (Wall Street Journal)
What to expect from Neuralink in 2025
More volunteers will get Elon Musk’s brain implant, but don’t expect a product soon. (MIT Technology Review)
A former fact-checking outlet for Meta signed a new deal to help train AI models
Meta paid media outlets like Agence France-Presse for years to do fact checking on its platforms. Since Meta announced it would shutter those programs, Europe’s leading AI company, Mistral, has signed a deal with AFP to use some of its content in its AI models. (Financial Times)
OpenAI’s AI reasoning model “thinks” in Chinese sometimes, and no one really knows why
While it comes to its response, the model often switches to Chinese, perhaps a reflection of the fact that many data labelers are based in China. (Tech Crunch)