Join our daily and weekly newsletters for the latest updates and exclusive content on industry-leading AI coverage. Learn More
A Brooklyn-based startup is taking aim at one of the most notorious pain points in the world of artificial intelligence and data analytics: the painstaking process of data preparation.
Structify emerged from stealth mode today, announcing its public launch alongside $4.1 million in seed funding led by Bain Capital Ventures, with participation from 8VC, Integral Ventures and strategic angel investors.
The company’s platform uses a proprietary visual language model called DoRa to automate the gathering, cleaning, and structuring of data — a process that typically consumes up to 80% of data scientists’ time, according to industry surveys.
“The volume of information available today has absolutely exploded,” said Ronak Gandhi, co-founder of Structify, in an exclusive interview with VentureBeat. “We’ve hit a major inflection point in data availability, which is both a blessing and a curse. While we have unprecedented access to information, it remains largely inaccessible because it’s so difficult to convert into the right format for making meaningful business decisions.”
Structify’s approach reflects a growing industry-wide focus on solving what data experts call “the data preparation bottleneck.” Gartner research indicates that inadequate data preparation remains one of the primary obstacles to successful AI implementation, with four of five businesses lacking the data foundations necessary to fully capitalize on generative AI.
How AI-powered data transformation is unlocking hidden business intelligence at scale
At its core, Structify allows users to create custom datasets by specifying the data schema, selecting sources, and deploying AI agents to extract that data. The platform can handle everything from SEC filings and LinkedIn profiles to news articles and specialized industry documents.
What sets Structify apart, according to Gandhi, is their in-house model DoRa, which navigates the web like a human would.
“It’s super high-quality. It navigates and interacts with stuff just like a person would,” Gandhi explained. “So we’re talking about human quality — that’s the first and foremost center of the principles behind DoRa. It reads the internet the way a human would.”
This approach allows Structify to support a free tier, which Gandhi believes will help democratize access to structured data.
“The way in which you think about data now is, it’s this really precious object,” Gandhi said. “This really precious thing that you spend so much time finagling and getting and wrestling around, and when you have it, you’re like, ‘Oh, if someone was to delete it, I would cry.’”
Structify’s vision is to “commoditize data” — making it something that can be easily recreated if lost.
From finance to construction: How businesses are deploying custom datasets to solve industry-specific challenges
The company has already seen adoption across multiple sectors. Finance teams use it to extract information from pitch decks, construction companies turn complex geotechnical documents into readable tables, and sales teams gather real-time organizational charts for their accounts.
Slater Stich, partner at Bain Capital Ventures, highlighted this versatility in the funding announcement: “Every company I’ve ever worked with has a handful of data sources that are both extremely important and a huge pain to work with, whether that’s figures buried in PDFs, scattered across hundreds of web pages, hidden behind an enterprise SOAP API, etc.”
The diversity of Structify’s early customer base reflects the universal nature of data preparation challenges. According to TechTarget research, data preparation typically involves a series of labor-intensive steps: collection, discovery, profiling, cleansing, structuring, transformation, and validation — all before any actual analysis can begin.
Why human expertise remains crucial for AI accuracy: Inside Structify’s ‘quadruple verification’ system
A key differentiator for Structify is its “quadruple verification” process, which combines AI with human oversight. This approach addresses a critical concern in AI development: ensuring accuracy.
“Whenever a user sees something that’s suspicious, or we identify some data as potentially suspicious, we can send it to an expert in that specific use case,” Gandhi explained. “That expert can act in the same way as [DoRa], navigate to the right piece of information, extract it, save it, and then verify if it’s right.”
This process not only corrects the data but also creates training examples that improve the model’s performance over time, especially in specialized domains like construction or pharmaceutical research.
“Those things are so messy,” Gandhi noted. “I never thought in my life I would have a strong understanding of geology. But there we are, and that, I think, is a huge strength – being able to learn from these experts and put it directly into DoRa.”
As data extraction tools become more powerful, privacy concerns inevitably arise. Structify has implemented safeguards to address these issues.
“We don’t do any authentication, anything that required a login, anything that requires you to go behind some sense of information – our agent doesn’t do that because that’s a privacy concern,” Gandhi said.
The company also prioritizes transparency by providing direct sourcing information. “If you’re interested in learning more about a particular piece of information, you go directly to that content and see it, as opposed to kind of legacy providers where it’s this black box.”
Structify enters a competitive landscape that includes both established players and other startups addressing various aspects of the data preparation challenge. Companies like Alteryx, Informatica, Microsoft, and Tableau all offer data preparation capabilities, while several specialists have been acquired in recent years.
What differentiates Structify, according to CEO Alex Reichenbach, is its combination of speed and accuracy. A recent LinkedIn post by Reichenbach claimed they had sped up their agent “10x while cutting cost ~16x” through model optimization and infrastructure improvements.
The company’s launch comes amid growing interest in AI-powered data automation. According to a TechTarget report, automating data preparation “is frequently cited as one of the major investment areas for data and analytics teams,” with augmented data preparation capabilities becoming increasingly important.
How frustrating data preparation experiences inspired two friends to revolutionize the industry
For Gandhi, Structify addresses problems he faced firsthand in previous roles.
“The big thing about the founding story of Structify is it’s both kind of a personal and a professional thing,” Gandhi recalled. “I was telling [Alex] about the time that I was working as a data analyst and doing ops and consulting, preparing these really niche, bespoke data sets for clients — lists of all the fitness influencers and their following metrics, lists of companies and what jobs they’re posting, museums on the East Coast… I was spending a lot of time doing manually curating them, scraping, data entry, all this stuff.”
The inability to quickly iterate from idea to dataset was particularly frustrating. “What got me was that you couldn’t iterate and kind of go from idea to data set in a quick fashion,” Gandhi said.
His co-founder, Alex Reichenbach, encountered similar challenges while working at an investment bank, where data quality issues hampered efforts to build models on top of structured datasets.
How Structify plans to use its $4.1 million seed funding to transform enterprise data preparation
With the new funding, Structify plans to grow its technical team and establish itself as “the go-to data tool across industries.” The company currently offers both free and paid tiers, with enterprise options for those needing advanced features like on-premise deployment or highly specialized data extraction.
As more companies invest in AI initiatives, the importance of high-quality, structured data will only increase. A recent MIT Technology Review Insights report found that four out of five businesses aren’t ready to capitalize on generative AI because of poor data foundations.
For Gandhi and the Structify team, solving this fundamental challenge could unlock significant value across industries.
“The fact that you can even imagine a world which creating data sets is iterative is kind of mind boggling for a lot of our users,” Gandhi said. “At the end of the day, the pitch is about being able to have this control and customizability.”
Daily insights on business use cases with VB Daily
If you want to impress your boss, VB Daily has you covered. We give you the inside scoop on what companies are doing with generative AI, from regulatory shifts to practical deployments, so you can share insights for maximum ROI.
Read our Privacy Policy
Thanks for subscribing. Check out more VB newsletters here.
An error occured.
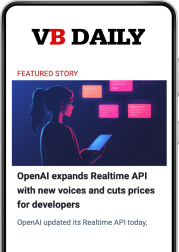