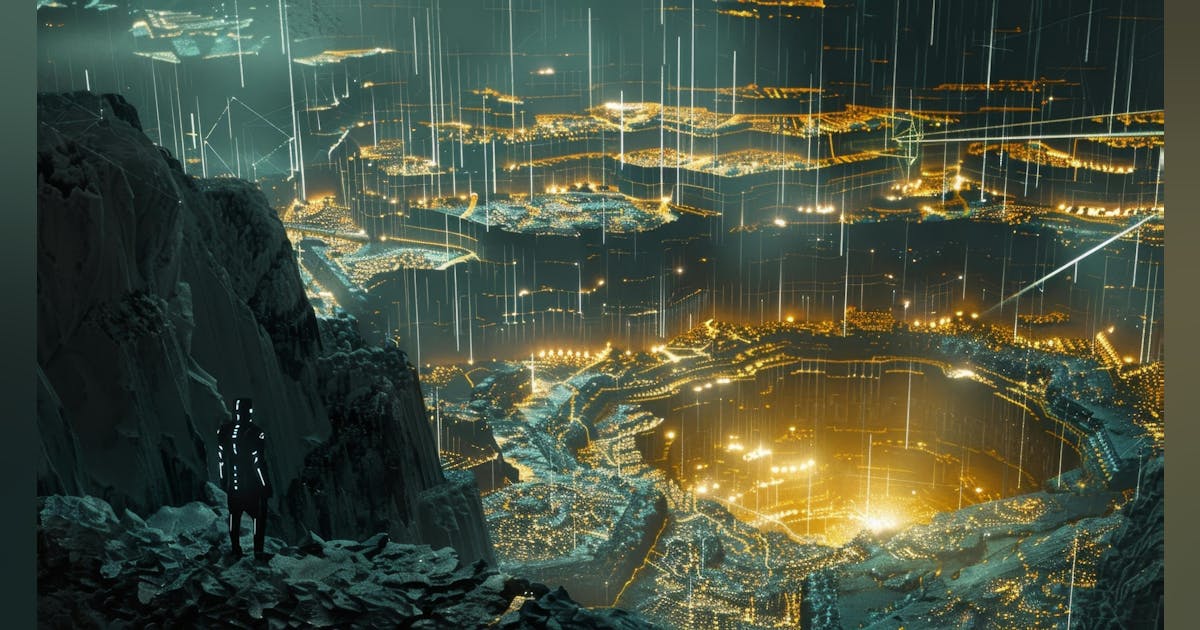
In 1849, the discovery of gold in California ignited a frenzy, drawing prospectors from around the world in pursuit of quick fortune. While few struck it rich digging and sifting dirt, a different class of entrepreneurs quietly prospered: those who supplied the miners with the tools of the trade. From picks and shovels to tents and provisions, these providers became indispensable to the gold rush, profiting handsomely regardless of who found gold.
Today, a new gold rush is underway, in pursuit of artificial intelligence. And just like the days of yore, the real fortunes may lie not in the gold itself, but in the infrastructure and equipment that enable its extraction. This is where neocloud players and chipmakers are positioned, representing themselves as the fundamental enablers of the AI revolution.
Neoclouds: The Essential Tools and Implements of AI Innovation
The AI boom has sparked a frenzy of innovation, investment, and competition. From generative AI applications like ChatGPT to autonomous systems and personalized recommendations, AI is rapidly transforming industries. Yet, behind every groundbreaking AI model lies an unsung hero: the infrastructure powering it. Enter neocloud providers—the specialized cloud platforms delivering the GPU horsepower that fuels AI’s meteoric rise. Let’s examine how neoclouds represent the “picks and shovels” of the AI gold rush, used for extracting the essential backbone of AI innovation.
Neoclouds are emerging as indispensable players in the AI ecosystem, offering tailored solutions for compute-intensive workloads such as training large language models (LLMs) and performing high-speed inference. Unlike traditional hyperscalers (e.g., AWS, Azure, Google Cloud), which cater to a broad range of use cases, neoclouds focus exclusively on optimizing infrastructure for AI and machine learning applications. This specialization allows them to deliver superior performance at a lower cost, making them the go-to choice for startups, enterprises, and research institutions alike.
The analogy to historical gold rushes is striking: just as miners relied on suppliers of picks and shovels to extract value from the earth, today’s AI pioneers depend on neocloud providers and chipmakers to access the computational resources needed to unlock insights and drive innovation. Neoclouds don’t compete in creating AI applications themselves; instead, they profit by enabling others to do so. This business model positions them as foundational players in the AI economy—profiting regardless of which companies or applications ultimately dominate the market.
Why Neoclouds Are Surging: Four Key Advantages
As AI development accelerates, the demand for compute is outpacing even the hyperscale cloud’s capacity to deliver. Training a foundation model like GPT-4 can require tens of thousands of GPUs running continuously for weeks—something traditional providers weren’t architected to support at scale. Enter the neoclouds: leaner, purpose-built platforms designed to meet the needs of modern AI workloads with greater precision and speed.
Here’s how they’re closing the gap:
1. Specialized Hardware
Neocloud providers are laser-focused on providing access to the newest and most powerful GPUs—often before hyperscalers can make them widely available. NVIDIA’s H100 and A100 accelerators, crucial for training and inference, are the cornerstone of these platforms. Many neoclouds go a step further, offering liquid-cooled racks, ultra-low-latency interconnects, and AI-specific storage tiers designed to keep pace with multi-petabyte datasets. For cutting-edge AI labs and fast-moving startups, this means the difference between weeks and months in development timelines.
2. Bare-Metal Performance
By eliminating the virtualization layers common in general-purpose clouds, neoclouds give users direct access to raw compute power. This bare-metal approach reduces latency and avoids the “noisy neighbor” problem, enabling highly deterministic performance—crucial when fine-tuning large language models or orchestrating tightly coupled GPU workloads. For teams pushing the edge of performance, every clock cycle matters, and neoclouds are delivering those cycles unfiltered.
3. Scalability on Demand
AI R&D is rarely linear. One month you’re iterating on small models, and the next you’re scaling to train a 70-billion-parameter transformer. Neocloud infrastructure is designed to expand and contract with those demands—supporting everything from a few nodes to full-scale superclusters. Unlike traditional clouds, which often impose capacity planning constraints or quotas, neoclouds thrive on elasticity, provisioning capacity dynamically and often within hours rather than weeks.
4. Cost Efficiency with Purpose-Built Pricing
Where hyperscalers often price GPU instances at a premium—factoring in legacy overhead and multi-tenant complexity—neoclouds keep things lean. Many operate with thinner margins and lower operational complexity, translating to significantly lower costs per training hour. Providers like Lambda, Run:ai, and Voltage Park offer transparent, workload-specific pricing that aligns with actual usage. High utilization rates and tailored provisioning models keep costs in check, making neoclouds especially appealing for startups and research groups running on grant cycles or VC runway.
These advantages make neoclouds invaluable not only for startups with limited budgets but also for established enterprises seeking to accelerate their AI initiatives.
Resilience at Scale: Why Neoclouds May Outlast the AI Hype Cycle
Investing in neoclouds offers a unique opportunity to participate in the AI boom without betting on specific applications or platforms. The rapid pace of innovation means that today’s leading AI models could be eclipsed by new breakthroughs tomorrow. However, regardless of which technologies prevail, the need for robust infrastructure will remain constant.
This dynamic mirrors historical gold rushes, where equipment suppliers thrived even as individual miners faced uncertainty. By providing essential tools for AI development, neocloud providers are positioned to benefit from sustained demand across diverse industries—from healthcare and finance to entertainment and logistics.
As the AI gold rush continues, neoclouds are poised to play an increasingly central role in shaping its trajectory. Their ability to deliver cost-effective, high-performance infrastructure makes them critical enablers of innovation. At the same time, their business model—focused on empowering others rather than competing directly—ensures they remain indispensable partners in the AI ecosystem.
Looking forward, neoclouds face challenges such as supply chain constraints for GPUs and competition from hyperscalers attempting to close the performance gap. However, their agility and specialization give them a distinct edge in navigating these hurdles. In many ways, they represent the future of cloud computing: leaner, faster, and more focused on solving specific problems.
As investors and enterprises seek ways to capitalize on AI’s transformative potential, neoclouds offer a compelling proposition—one that promises steady growth amid the chaos of rapid technological change.
Economic Disruption: How Neoclouds Are Redefining Cost and Performance
The emergence of neoclouds is causing a significant economic disruption in the AI infrastructure landscape. Unlike traditional hyperscalers that offer a broad range of services, neocloud providers concentrate on delivering optimized price-performance specifically for AI workloads. This specialization translates into several key advantages: higher GPU utilization rates, bare-metal access, and the application of deep, specialist expertise. These elements combine to create a compelling economic proposition for AI developers and enterprises.
The numbers speak for themselves. Neoclouds are achieving significant cost reductions, with reports from Uptime Institute indicating as much as 66% savings on GPU instances when compared to major hyperscalers. This substantial difference stems from the ability to maximize the use of expensive GPU resources and minimize overhead. For organizations running large-scale AI training or inference tasks, this can lead to considerable savings in operational expenses.
The efficiencies introduced by neoclouds are reshaping the overall economics of AI development. As models grow in complexity and require more compute power, the cost of training and deploying them has become a major barrier. By lowering these costs, neoclouds make it feasible for a wider array of organizations – from startups to established corporations – to engage in AI initiatives. This democratization of AI resources has the potential to accelerate innovation across diverse sectors, allowing more companies to harness the power of AI without breaking the bank.
Advancing Coopetition Between Neocloud Providers and Hyperscalers
The relationship between neocloud providers and traditional hyperscalers is increasingly defined by a complex blend of competition and collaboration. As the demand for AI infrastructure surges, both camps are vying for dominance in the lucrative GPU cloud market, yet their interactions are far from zero-sum, resulting in a competitive, yet symbiotic, market landscape.
Neoclouds have carved out a niche by specializing in GPU-accelerated infrastructure tailored for AI and machine learning workloads. Their agility, focus, and deep understanding of AI developers’ needs allow them to offer cost-effective, high-performance solutions that challenge the broader, premium-priced offerings of hyperscalers. While hyperscalers benefit from vast economies of scale and integrated ecosystems, their diversified business models and high-margin pricing strategies on their cloud businesses often result in higher prices for AI-specific resources.
Despite this competition, the two groups are increasingly intertwined. Neoclouds often position themselves not as direct competitors, but as complementary partners within enterprise multi-cloud strategies. For example, an organization might use a neocloud to train a large language model, then deploy it on a hyperscaler’s platform for inference and integration with other services. This approach allows enterprises to optimize for both performance and cost, leveraging the strengths of each provider.
Investment, Partnership, and Multi-Cloud Integration
The interplay between neoclouds and hyperscalers is further complicated by growing investment and partnership activity. Hyperscalers are not only competing with neoclouds but also investing in them and, in some cases, becoming their customers. A prominent example is Microsoft’s $10 billion commitment to CoreWeave to secure access to specialized GPU infrastructure through 2029. Such deals highlight the recognition by hyperscalers of the unique value neoclouds bring to the AI infrastructure ecosystem.
For enterprises, this dynamic is accelerating the adoption of multi-cloud strategies. By integrating neoclouds into their cloud portfolios, organizations can avoid vendor lock-in, optimize for specific workloads, and ensure access to scarce GPU resources. However, this also introduces new complexities, as enterprises must now manage interoperability and data movement across increasingly fragmented cloud environments.
Looking forward, the evolving relationship between neoclouds and hyperscalers increases the prospects (and pricing) of further M&A activity across key players. As neoclouds grow in scale and strategic importance, it is likely that some will be acquired by hyperscalers seeking to bolster their AI infrastructure capabilities and maintain competitive advantage. Such consolidation will reshape the market’s structure, potentially accelerating innovation through deeper integration, but also raising questions about pricing power and the pace of future disruption.
High Stakes Require High Investment
The capital intensity of neoclouds is staggering. Building and maintaining these specialized infrastructure platforms requires massive investments in GPUs, networking equipment, and data center facilities. To finance this expansion, neoclouds have tapped into both equity and debt markets, with some pioneering the use of GPU assets as collateral for loans. This approach allows them to leverage their hardware investments more efficiently, but also exposes them to the risk of depreciating GPU values and limited liquidity.
The market is also seeing large supply chain vulnerabilities, and rapid equipment obsolescence, increasing the risk of relying too heavily on any one provider. The rapid pace of innovation in chip design presents both opportunities and challenges. While new generations of GPUs promise increased performance and efficiency, they also render older hardware obsolete on extremely short timelines.
This creates a constant pressure to upgrade infrastructure, potentially straining finances and exacerbating supply chain vulnerabilities. Geopolitical factors and manufacturing bottlenecks can further disrupt the supply of GPUs, as we are seeing with recent tariffs affecting NVIDIA, thus impacting neocloud providers’ abilities to meet growing demand.
The Chipmaker Landscape: Navigating the Silicon Battleground of the Neocloud Era
The neocloud boom is redefining the data center ecosystem, driven by billions in venture capital and a red-hot GPU-backed debt market that’s rewriting the rules of infrastructure financing. But while the capital is flowing fast, the underlying hardware story is anything but straightforward. Supply chain constraints, hardware churn, and the specter of over-leverage hang over the sector as neocloud builders sprint to scale. At the heart of this high-stakes race sit the chipmakers — the true power brokers of the AI infrastructure gold rush.
NVIDIA holds the pole position, having effectively set the standard for AI compute with its high-performance GPUs and the proprietary CUDA software stack that developers now treat as foundational. The company’s dominance isn’t just about raw silicon; it’s about controlling the ecosystem. But as demand for AI infrastructure skyrockets, so do concerns about supply bottlenecks, pricing leverage, and the systemic risks of depending too heavily on a single vendor.
Enter AMD and Intel, both aggressively positioning themselves as viable alternatives. AMD’s Instinct accelerators have made meaningful headway, especially among hyperscalers and research labs looking for more open, programmable environments. Its embrace of open-source software and its tight integration across CPU and GPU workloads gives it an edge in environments where flexibility and long-term value matter.
Intel, meanwhile, is betting on a diversified portfolio and a vertically integrated approach. With CPUs, discrete GPUs, and dedicated AI accelerators (via Habana Labs), Intel is aiming to meet the market wherever the workload lands — from training massive models to powering real-time inference at the edge. Its growing software investments signal a deeper push to win developer mindshare, not just silicon sockets.
What’s increasingly clear is that chipmakers now play a strategic role that transcends component supply. Their influence touches everything from AI model optimization to deployment timelines and total cost of ownership. In a capital-intensive market where seconds of inference time and watts per rack can make or break a business model, silicon choices aren’t just technical — they’re existential.
For neocloud operators, the path forward demands architectural agility. Betting solely on NVIDIA may win short-term performance, but long-term resilience will require multi-vendor strategies that hedge against market shocks, broaden workload compatibility, and enhance buyer leverage. The most successful neocloud platforms will be those that understand chipmakers not just as suppliers, but as strategic partners — and occasionally, as competitive threats.
As the AI era matures, the chipmaker battleground will increasingly shape who wins the neocloud race — and who gets left behind in the silicon stampede.
Which Provides the Winning Competitive Edge: Specialization or Scale?
The rise of neoclouds has been driven by their ability to deliver specialized, high-performance infrastructure tailored for AI workloads. However, the question remains: are these advantages sustainable in the long term, or do they merely represent a transitional phase before hyperscalers catch up? For neoclouds to maintain a competitive edge, there are many potential scenarios to consider.
The Bull Case: Persistent Barriers to Entry
The argument for neoclouds’ long-term viability rests on several key factors:
Technical Complexity: Building and managing AI-optimized infrastructure requires deep expertise in GPU architecture, networking, and software. Neoclouds have cultivated this expertise over time, creating a barrier to entry that is difficult for hyperscalers to replicate quickly.
Specialization: Neoclouds focus solely on AI workloads, allowing them to optimize their infrastructure and services for the specific needs of AI developers. This specialization translates into superior performance and cost-efficiency compared to the more generalized offerings of hyperscalers.
Agility: Neoclouds tend to be smaller and more agile than hyperscalers, enabling them to adapt quickly to changing market conditions and emerging technologies. This agility is particularly valuable in the rapidly evolving field of AI.
The Bear Case: Margin Compression and Scale Advantages
Despite many advantages, neoclouds face significant challenges from hyperscalers:
Margin Compression: As hyperscalers invest more heavily in AI infrastructure and refine their offerings, they may be able to erode the price advantage currently enjoyed by neoclouds. Hyperscalers’ scale economies and ability to cross-subsidize AI services with other cloud offerings could put significant pressure on neocloud margins.
Scale Advantages: Hyperscalers possess massive economies of scale, allowing them to procure hardware at lower prices and invest more heavily in R&D. This scale advantage could enable them to leapfrog neoclouds in terms of performance and innovation.
Ecosystem Integration: Hyperscalers offer tightly integrated ecosystems of cloud services, making it easier for customers to build and deploy AI applications. Neoclouds may struggle to match this level of integration, particularly for enterprises that rely on a wide range of cloud services.
Scenarios for the Future
The future of the neocloud market is uncertain, but several scenarios are possible:
Coexistence: Neoclouds and hyperscalers coexist, with each catering to different segments of the market. Neoclouds focus on specialized AI workloads and customers who prioritize performance and cost-efficiency, while hyperscalers cater to enterprises seeking a broader range of cloud services and ecosystem integration.
Market Consolidation: Hyperscalers acquire leading neoclouds to bolster their AI infrastructure capabilities and gain access to specialized expertise. This scenario could lead to greater integration and innovation, but also raise concerns about pricing power and market competition.
Disruption: Neoclouds continue to innovate and disrupt the market, challenging the dominance of hyperscalers and attracting a growing share of AI workloads. This scenario would require neoclouds to overcome challenges related to scale, ecosystem integration, and capital access.
Ultimately, the long-term success of neoclouds will depend on their ability to differentiate themselves from hyperscalers, innovate continuously, and adapt to the rapidly evolving needs of the AI community.
Making AI More Accessible: Who’s Using Neoclouds—and Why It Matters
The neocloud market represents a dynamic and essential force driving innovation in the age of AI. By specializing in high-performance, cost-effective infrastructure, neocloud providers are not only enabling the AI revolution, but also reshaping the economics of cloud computing.
Whether as “picks and shovels” suppliers to the AI gold rush, or as competitive partners alongside hyperscalers, neoclouds are proving their enduring value in a rapidly evolving technological landscape. And as they navigate the challenges of scale, financing, and chipmaker dependencies, the neoclouds are poised to continue pushing the boundaries of what’s possible in AI, fostering a more accessible and innovative future for all.