Join our daily and weekly newsletters for the latest updates and exclusive content on industry-leading AI coverage. Learn More
January 2025 shook the AI landscape. The seemingly unstoppable OpenAI and the powerful American tech giants were shocked by what we can certainly call an underdog in the area of large language models (LLMs). DeepSeek, a Chinese firm not on anyone’s radar, suddenly challenged OpenAI. It is not that DeepSeek-R1 was better than the top models from American giants; it was slightly behind in terms of the benchmarks, but it suddenly made everyone think about the efficiency in terms of hardware and energy usage.
Given the unavailability of the best high-end hardware, it seems that DeepSeek was motivated to innovate in the area of efficiency, which was a lesser concern for larger players. OpenAI has claimed they have evidence suggesting DeepSeek may have used their model for training, but we have no concrete proof to support this. So, whether it is true or it’s OpenAI simply trying to appease their investors is a topic of debate. However, DeepSeek has published their work, and people have verified that the results are reproducible at least on a much smaller scale.
But how could DeepSeek attain such cost-savings while American companies could not? The short answer is simple: They had more motivation. The long answer requires a little bit more of a technical explanation.
DeepSeek used KV-cache optimization
One important cost-saving for GPU memory was optimization of the Key-Value cache used in every attention layer in an LLM.
LLMs are made up of transformer blocks, each of which comprises an attention layer followed by a regular vanilla feed-forward network. The feed-forward network conceptually models arbitrary relationships, but in practice, it is difficult for it to always determine patterns in the data. The attention layer solves this problem for language modeling.
The model processes texts using tokens, but for simplicity, we will refer to them as words. In an LLM, each word gets assigned a vector in a high dimension (say, a thousand dimensions). Conceptually, each dimension represents a concept, like being hot or cold, being green, being soft, being a noun. A word’s vector representation is its meaning and values according to each dimension.
However, our language allows other words to modify the meaning of each word. For example, an apple has a meaning. But we can have a green apple as a modified version. A more extreme example of modification would be that an apple in an iPhone context differs from an apple in a meadow context. How do we let our system modify the vector meaning of a word based on another word? This is where attention comes in.
The attention model assigns two other vectors to each word: a key and a query. The query represents the qualities of a word’s meaning that can be modified, and the key represents the type of modifications it can provide to other words. For example, the word ‘green’ can provide information about color and green-ness. So, the key of the word ‘green’ will have a high value on the ‘green-ness’ dimension. On the other hand, the word ‘apple’ can be green or not, so the query vector of ‘apple’ would also have a high value for the green-ness dimension. If we take the dot product of the key of ‘green’ with the query of ‘apple,’ the product should be relatively large compared to the product of the key of ‘table’ and the query of ‘apple.’ The attention layer then adds a small fraction of the value of the word ‘green’ to the value of the word ‘apple’. This way, the value of the word ‘apple’ is modified to be a little greener.
When the LLM generates text, it does so one word after another. When it generates a word, all the previously generated words become part of its context. However, the keys and values of those words are already computed. When another word is added to the context, its value needs to be updated based on its query and the keys and values of all the previous words. That’s why all those values are stored in the GPU memory. This is the KV cache.
DeepSeek determined that the key and the value of a word are related. So, the meaning of the word green and its ability to affect greenness are obviously very closely related. So, it is possible to compress both as a single (and maybe smaller) vector and decompress while processing very easily. DeepSeek has found that it does affect their performance on benchmarks, but it saves a lot of GPU memory.
DeepSeek applied MoE
The nature of a neural network is that the entire network needs to be evaluated (or computed) for every query. However, not all of this is useful computation. Knowledge of the world sits in the weights or parameters of a network. Knowledge about the Eiffel Tower is not used to answer questions about the history of South American tribes. Knowing that an apple is a fruit is not useful while answering questions about the general theory of relativity. However, when the network is computed, all parts of the network are processed regardless. This incurs huge computation costs during text generation that should ideally be avoided. This is where the idea of the mixture-of-experts (MoE) comes in.
In an MoE model, the neural network is divided into multiple smaller networks called experts. Note that the ‘expert’ in the subject matter is not explicitly defined; the network figures it out during training. However, the networks assign some relevance score to each query and only activate the parts with higher matching scores. This provides huge cost savings in computation. Note that some questions need expertise in multiple areas to be answered properly, and the performance of such queries will be degraded. However, because the areas are figured out from the data, the number of such questions is minimised.
The importance of reinforcement learning
An LLM is taught to think through a chain-of-thought model, with the model fine-tuned to imitate thinking before delivering the answer. The model is asked to verbalize its thought (generate the thought before generating the answer). The model is then evaluated both on the thought and the answer, and trained with reinforcement learning (rewarded for a correct match and penalized for an incorrect match with the training data).
This requires expensive training data with the thought token. DeepSeek only asked the system to generate the thoughts between the tags and and to generate the answers between the tags and . The model is rewarded or penalized purely based on the form (the use of the tags) and the match of the answers. This required much less expensive training data. During the early phase of RL, the model tried generated very little thought, which resulted in incorrect answers. Eventually, the model learned to generate both long and coherent thoughts, which is what DeepSeek calls the ‘a-ha’ moment. After this point, the quality of the answers improved quite a lot.
DeepSeek employs several additional optimization tricks. However, they are highly technical, so I will not delve into them here.
Final thoughts about DeepSeek and the larger market
In any technology research, we first need to see what is possible before improving efficiency. This is a natural progression. DeepSeek’s contribution to the LLM landscape is phenomenal. The academic contribution cannot be ignored, whether or not they are trained using OpenAI output. It can also transform the way startups operate. But there is no reason for OpenAI or the other American giants to despair. This is how research works — one group benefits from the research of the other groups. DeepSeek certainly benefited from the earlier research performed by Google, OpenAI and numerous other researchers.
However, the idea that OpenAI will dominate the LLM world indefinitely is now very unlikely. No amount of regulatory lobbying or finger-pointing will preserve their monopoly. The technology is already in the hands of many and out in the open, making its progress unstoppable. Although this may be a little bit of a headache for the investors of OpenAI, it’s ultimately a win for the rest of us. While the future belongs to many, we will always be thankful to early contributors like Google and OpenAI.
Debasish Ray Chawdhuri is senior principal engineer at Talentica Software.
Daily insights on business use cases with VB Daily
If you want to impress your boss, VB Daily has you covered. We give you the inside scoop on what companies are doing with generative AI, from regulatory shifts to practical deployments, so you can share insights for maximum ROI.
Read our Privacy Policy
Thanks for subscribing. Check out more VB newsletters here.
An error occured.
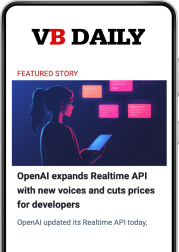