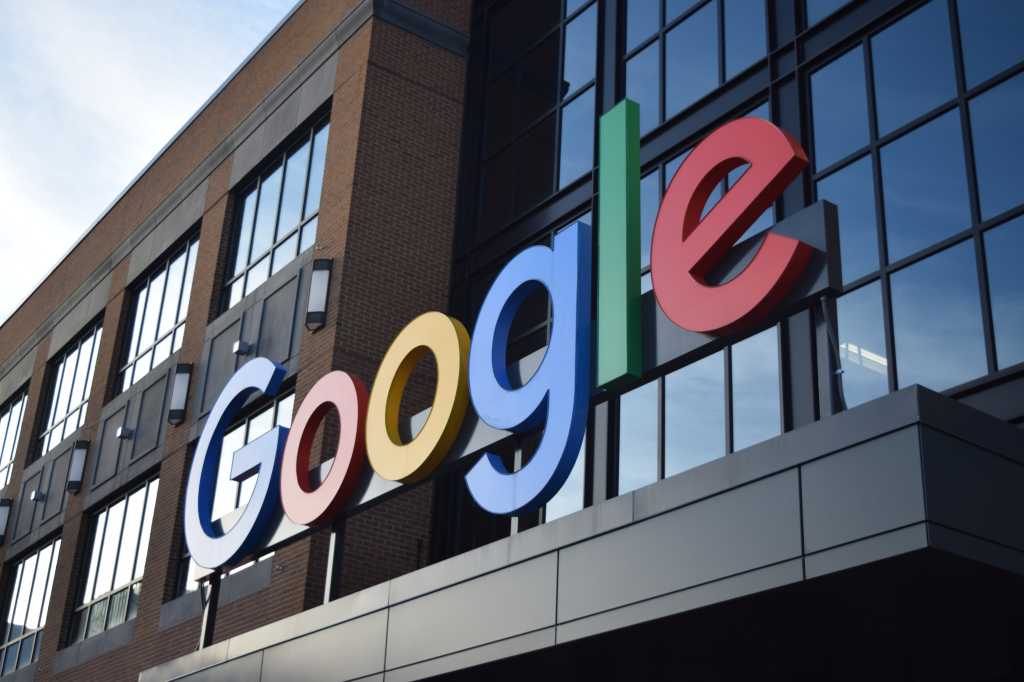
“We are witnessing a divergence in hyperscaler strategy,” noted Abhivyakti Sengar, practice director at Everest Group. “Google is doubling down on global, AI-first scale; Microsoft is signaling regional optimization and selective restraint. For enterprises, this changes the calculus.”
Meanwhile, OpenAI is reportedly exploring building its own data center infrastructure to reduce reliance on cloud providers and increase its computing capabilities.
Shifting enterprise priorities
For CIOs and enterprise architects, these divergent infrastructure approaches present new considerations when planning AI deployments. Organizations must now evaluate not just immediate availability, but long-term infrastructure alignment with their AI roadmaps.
“Enterprise cloud strategies for AI are no longer just about picking a hyperscaler — they’re increasingly about workload sovereignty, GPU availability, latency economics, and AI model hosting rights,” said Sanchit Gogia, CEO and chief analyst at Greyhound Research.
According to Greyhound’s research, 61% of large enterprises now prioritize “AI-specific procurement criteria” when evaluating cloud providers — up from just 24% in 2023. These criteria include model interoperability, fine-tuning costs, and support for open-weight alternatives.
The rise of multicloud strategies
As hyperscalers pursue different approaches to AI infrastructure, enterprise IT leaders are increasingly adopting multicloud strategies as a risk mitigation measure.