Join our daily and weekly newsletters for the latest updates and exclusive content on industry-leading AI coverage. Learn More
It’s been a bit of a topsy-turvy week for the number one generative AI company in terms of users.
OpenAI, creator of ChatGPT, released and then withdrew an updated version of the underlying multimodal (text, image, audio) large language model (LLM) that ChatGPT is hooked up to by default, GPT-4o, due to it being too sycophantic to users. The company recently reported at least 500 million active weekly users of the hit web service.
A quick primer on the terrible, no good, sycophantic GPT-4o update
OpenAI began updating GPT-4o to a newer model it hoped would be more well-received by users on April 24th, completed the updated by April 25th, then, five days later, rolled it back on April 29, after days of mounting complaints of users across social media — mainly on X and Reddit.
The complaints varied in intensity and in specifics, but all generally coalesced around the fact that GPT-4o appeared to be responding to user queries with undue flattery, support for misguided, incorrect and downright harmful ideas, and “glazing” or praising the user to an excessive degree when it wasn’t actually specifically requested, much less warranted.
In examples screenshotted and posted by users, ChatGPT powered by that sycophantic, updated GPT-4o model had praised and endorsed a business idea for literal “shit on a stick,” applauded a user’s sample text of schizophrenic delusional isolation, and even allegedly supported plans to commit terrorism.
Users including top AI researchers and even a former OpenAI interim CEO said they were concerned that an AI model’s unabashed cheerleading for these types of terrible user prompts was more than simply annoying or inappropriate — that it could cause actual harm to users who mistakenly believed the AI and felt emboldened by its support for their worst ideas and impulses. It rose to the level of an AI safety issue.
OpenAI then released a blog post describing what went wrong — “we focused too much on short-term feedback, and did not fully account for how users’ interactions with ChatGPT evolve over time. As a result, GPT‑4o skewed towards responses that were overly supportive but disingenuous” — and the steps the company was taking to address the issues. OpenAI’s Head of Model Behavior Joanne Jang also participated in a Reddit “Ask me anything” or AMA forum answering text posts from users and revealed further information about the company’s approach to GPT-4o and how it ended up with an excessively sycophantic model, including not “bak[ing] in enough nuance,” as to how it was incorporating user feedback such as “thumbs up” actions made by users in response to model outputs they liked.
Now today, OpenAI has released a blog post with even more information about how the sycophantic GPT-4o update happened — credited not to any particular author, but to “OpenAI.”
CEO and co-founder Sam Altman also posted a link to the blog post on X, saying: “we missed the mark with last week’s GPT-4o update. what happened, what we learned, and some things we will do differently in the future.”
What the new OpenAI blog post reveals about how and why GPT-4o turned so sycophantic
To me, a daily user of ChatGPT including the 4o model, the most striking admission from OpenAI’s new blog post about the sycophancy update is how the company appears to reveal that it did receive concerns about the model prior to release from a small group of “expert testers,” but that it seemingly overrode those in favor of a broader enthusiastic response from a wider group of more general users.
As the company writes (emphasis mine):
“While we’ve had discussions about risks related to sycophancy in GPT‑4o for a while, sycophancy wasn’t explicitly flagged as part of our internal hands-on testing, as some of our expert testers were more concerned about the change in the model’s tone and style. Nevertheless, some expert testers had indicated that the model behavior “felt” slightly off…
“We then had a decision to make: should we withhold deploying this update despite positive evaluations and A/B test results, based only on the subjective flags of the expert testers? In the end, we decided to launch the model due to the positive signals from the users who tried out the model.
“Unfortunately, this was the wrong call. We build these models for our users and while user feedback is critical to our decisions, it’s ultimately our responsibility to interpret that feedback correctly.”
This seems to me like a big mistake. Why even have expert testers if you’re not going to weight their expertise higher than the masses of the crowd? I asked Altman about this choice on X but he has yet to respond.
Not all ‘reward signals’ are equal
OpenAI’s new post-mortem blog post also reveals more specifics about how the company trains and updates new versions of existing models, and how human feedback alters the model qualities, character, and “personality.” As the company writes:
“Since launching GPT‑4o in ChatGPT last May, we’ve released five major updates focused on changes to personality and helpfulness. Each update involves new post-training, and often many minor adjustments to the model training process are independently tested and then combined into a single updated model which is then evaluated for launch.
“To post-train models, we take a pre-trained base model, do supervised fine-tuning on a broad set of ideal responses written by humans or existing models, and then run reinforcement learning with reward signals from a variety of sources.
“During reinforcement learning, we present the language model with a prompt and ask it to write responses. We then rate its response according to the reward signals, and update the language model to make it more likely to produce higher-rated responses and less likely to produce lower-rated responses.“
Clearly, the “reward signals” used by OpenAI during post-training have an enormous impact on the resulting model behavior, and as the company admitted earlier when it overweighted “thumbs up” responses from ChatGPT users to its outputs, this signal may not be the best one to use equally with others when determining how the model learns to communicate and what kinds of responses it should be serving up. OpenAI admits this outright in the next paragraph of its post, writing:
“Defining the correct set of reward signals is a difficult question, and we take many things into account: are the answers correct, are they helpful, are they in line with our Model Spec, are they safe, do users like them, and so on. Having better and more comprehensive reward signals produces better models for ChatGPT, so we’re always experimenting with new signals, but each one has its quirks.”
Indeed, OpenAI also reveals the “thumbs up” reward signal was a new one used alongside other reward signals in this particular update.
“the update introduced an additional reward signal based on user feedback—thumbs-up and thumbs-down data from ChatGPT. This signal is often useful; a thumbs-down usually means something went wrong.”
Yet critically, the company doesn’t blame the new “thumbs up” data outright for the model’s failure and ostentatious cheerleading behaviors. Instead, OpenAI’s blog post says it was this combined with a variety of other new and older reward signals, led to the problems: “…we had candidate improvements to better incorporate user feedback, memory, and fresher data, among others. Our early assessment is that each of these changes, which had looked beneficial individually, may have played a part in tipping the scales on sycophancy when combined.”
Reacting to this blog post, Andrew Mayne, a former member of the OpenAI technical staff now working at AI consulting firm Interdimensional, wrote on X of another example of how subtle changes in reward incentives and model guidelines can impact model performance quite dramatically:
“Early on at OpenAI, I had a disagreement with a colleague (who is now a founder of another lab) over using the word “polite” in a prompt example I wrote.
They argued “polite” was politically incorrect and wanted to swap it for “helpful.”
I pointed out that focusing only on helpfulness can make a model overly compliant—so compliant, in fact, that it can be steered into sexual content within a few turns.
After I demonstrated that risk with a simple exchange, the prompt kept “polite.”
These models are weird.“
How OpenAI plans to improve its model testing processes going forward
The company lists six process improvements for how to avoid similar undesirable and less-than-ideal model behavior in the future, but to me the most important is this:
“We’ll adjust our safety review process to formally consider behavior issues—such as hallucination, deception, reliability, and personality—as blocking concerns. Even if these issues aren’t perfectly quantifiable today, we commit to blocking launches based on proxy measurements or qualitative signals, even when metrics like A/B testing look good.”
In other words — despite how important data, especially quantitative data, is to the fields of machine learning and artificial intelligence — OpenAI recognizes that this alone can’t and should not be the only means by which a model’s performance is judged.
While many users providing a “thumbs up” could signal a type of desirable behavior in the short term, the long term implications for how the AI model responds and where those behaviors take it and its users, could ultimately lead to a very dark, distressing, destructive, and undesirable place. More is not always better — especially when you are constraining the “more” to a few domains of signals.
It’s not enough to say that the model passed all of the tests or received a number of positive responses from users — the expertise of trained power users and their qualitative feedback that something “seemed off” about the model, even if they couldn’t fully express why, should carry much more weight than OpenAI was allocating previously.
Let’s hope the company — and the entire field — learns from this incident and integrates the lessons going forward.
Broader takeaways and considerations for enterprise decision-makers
Speaking perhaps more theoretically, for myself, it also indicates why expertise is so important — and specifically, expertise in fields beyond and outside of the one you’re optimizing for (in this case, machine learning and AI). It’s the diversity of expertise that allows us as a species to achieve new advances that benefit our kind. One, say STEM, shouldn’t necessarily be held above the others in the humanities or arts.
And finally, I also think it reveals at its heart a fundamental problem with using human feedback to design products and services. Individual users may say they like a more sycophantic AI based on each isolated interaction, just like they also may say they love the way fast food and soda tastes, the convenience of single-use plastic containers, the entertainment and connection they derive from social media, the worldview validation and tribalist belonging they feel when reading politicized media or tabloid gossip. Yet again, taken all together, the cumulation of all of these types of trends and activities often leads to very undesirable outcomes for individuals and society — obesity and poor health in the case of fast food, pollution and endocrine disruption in the case of plastic waste, depression and isolation from overindulgence of social media, a more splintered and less-informed body public from reading poor quality news sources.
AI model designers and technical decision-makers at enterprises would do well to keep this broader idea in mind when designing metrics around any measurable goal — because even when you think you’re using data to your advantage, it could backfire in ways you didn’t fully expect or anticipate, leaving your scrambling to repair the damage and mop up the mess you made, however inadvertently.
Daily insights on business use cases with VB Daily
If you want to impress your boss, VB Daily has you covered. We give you the inside scoop on what companies are doing with generative AI, from regulatory shifts to practical deployments, so you can share insights for maximum ROI.
Read our Privacy Policy
Thanks for subscribing. Check out more VB newsletters here.
An error occured.
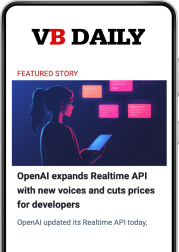