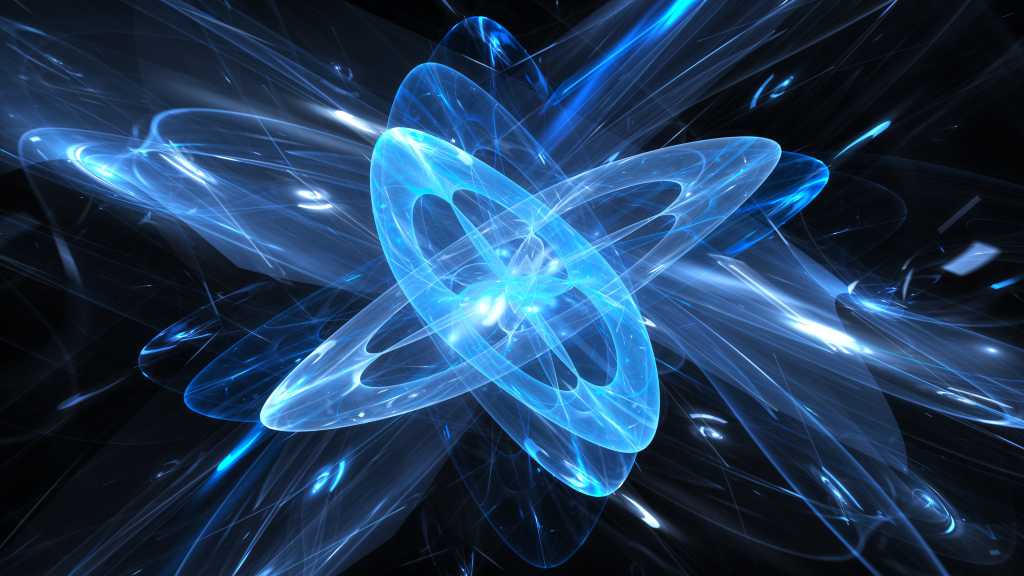
The RIKEN team, including Nori, Clemens Gneiting, and Yexiong Zeng, developed a deep learning method to optimize GKP states, making them easier to produce while maintaining robust error correction.
“Our AI-driven method fine-tunes the structure of GKP states, striking an optimal balance between resource efficiency and error resilience,” said Zeng in the statement. The results were striking. “The neural network achieved a much more efficient encoding than we had initially expected,” he said.
These optimized codes require fewer squeezed states and outperform traditional GKP codes, particularly in bosonic systems like superconducting cavities or photonics.
Vyshak cautioned that AI-optimized GKP codes excel in specific platforms but may not generalize across all quantum hardware. “Surface codes and LDPC codes remain more versatile and proven, especially in superconducting or trapped-ion systems,” he said. Still, RIKEN’s work significantly lowers the experimental barrier for certain architectures, accelerating progress toward practical quantum computing.
Global race for Quantum reliability
Quantum error correction is a critical focus worldwide, with researchers and industry leaders racing to overcome the challenges of qubit fragility. A December 2024 study on AI in QEC flagged its superiority over hand-crafted methods, especially as systems scale and error syndromes grow exponentially complex.
Vyshak emphasized that AI is becoming essential for managing the complexity of error correction at scale. “The volume and complexity of error syndromes in large quantum systems overwhelm traditional decoders,” he said. Neural networks and reinforcement learning adapt to dynamic noise patterns, optimize code parameters, and reduce processing bottlenecks, giving AI-driven solutions a competitive edge.