Introduction
Data science is undoubtedly one of the most fascinating fields today. Following significant breakthroughs in machine learning about a decade ago, data science has surged in popularity within the tech community. Each year, we witness increasingly powerful tools that once seemed unimaginable. Innovations such as the Transformer architecture, ChatGPT, the Retrieval-Augmented Generation (RAG) framework, and state-of-the-art Computer Vision models — including GANs — have had a profound impact on our world.
However, with the abundance of tools and the ongoing hype surrounding AI, it can be overwhelming — especially for beginners — to determine which skills to prioritize when aiming for a career in data science. Moreover, this field is highly demanding, requiring substantial dedication and perseverance.
The first three parts of this series outlined the necessary skills to become a data scientist in three key areas: math, software engineering, and machine learning. While knowledge of classical Machine Learning and neural network algorithms is an excellent starting point for aspiring data specialists, there are still many important topics in machine learning that must be mastered to work on more advanced projects.
This article will focus solely on the math skills necessary to start a career in Data Science. Whether pursuing this path is a worthwhile choice based on your background and other factors will be discussed in a separate article.
The importance of learning evolution of methods in machine learning
The section below provides information about the evolution of methods in natural language processing (NLP).
In contrast to previous articles in this series, I have decided to change the format in which I present the necessary skills for aspiring data scientists. Instead of directly listing specific competencies to develop and the motivation behind mastering them, I will briefly outline the most important approaches, presenting them in chronological order as they have been developed and used over the past decades in machine learning.
The reason is that I believe it is crucial to study these algorithms from the very beginning. In machine learning, many new methods are built upon older approaches, which is especially true for NLP and computer vision.
For example, jumping directly into the implementation details of modern large language models (LLMs) without any preliminary knowledge may make it very difficult for beginners to grasp the motivation and underlying ideas of specific mechanisms.
Given this, in the next two sections, I will highlight in bold the key concepts that should be studied.
# 04. NLP
Natural language processing (NLP) is a broad field that focuses on processing textual information. Machine learning algorithms cannot work directly with raw text, which is why text is usually preprocessed and converted into numerical vectors that are then fed into neural networks.
Before being converted into vectors, words undergo preprocessing, which includes simple techniques such as parsing, stemming, lemmatization, normalization, or removing stop words. After preprocessing, the resulting text is encoded into tokens. Tokens represent the smallest textual elements in a collection of documents. Generally, a token can be a part of a word, a sequence of symbols, or an individual symbol. Ultimately, tokens are converted into numerical vectors.
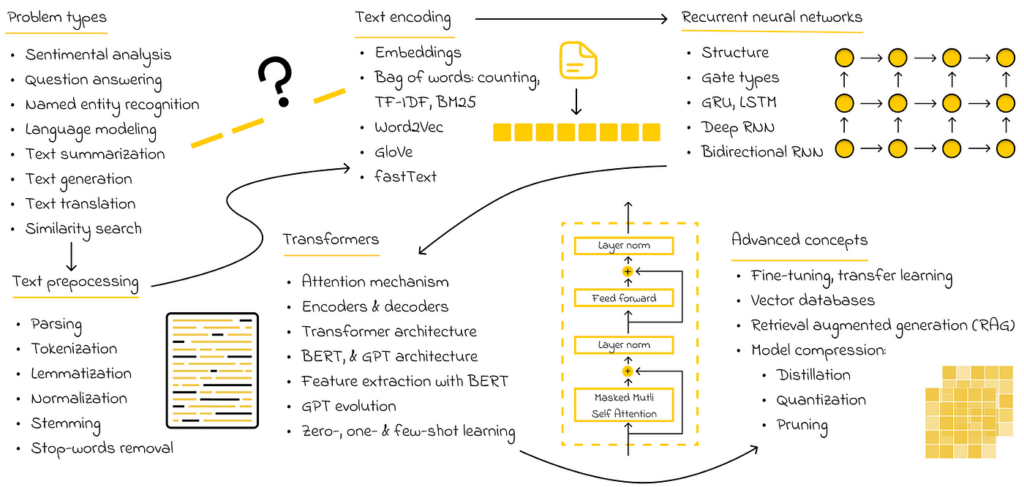
The bag of words method is the most basic way to encode tokens, focusing on counting the frequency of tokens in each document. However, in practice, this is usually not sufficient, as it is also necessary to account for token importance — a concept introduced in the TF-IDF and BM25 methods. While TF-IDF improves upon the naive counting approach of bag of words, researchers have developed a completely new approach called embeddings.
Embeddings are numerical vectors whose components preserve the semantic meanings of words. Because of this, embeddings play a crucial role in NLP, enabling input data to be trained or used for model inference. Additionally, embeddings can be used to compare text similarity, allowing for the retrieval of the most relevant documents from a collection.
Embeddings can also be used to encode other unstructured data, including images, audio, and videos.
As a field, NLP has been evolving rapidly over the last 10–20 years to efficiently solve various text-related problems. Complex tasks like text translation and text generation were initially addressed using recurrent neural networks (RNNs), which introduced the concept of memory, allowing neural networks to capture and retain key contextual information in long documents.
Although RNN performance gradually improved, it remained suboptimal for certain tasks. Moreover, RNNs are relatively slow, and their sequential prediction process does not allow for parallelization during training and inference, making them less efficient.
Additionally, the original Transformer architecture can be decomposed into two separate modules: BERT and GPT. Both of these form the foundation of the most state-of-the-art models used today to solve various NLP problems. Understanding their principles is valuable knowledge that will help learners advance further when studying or working with other large language models (LLMs).
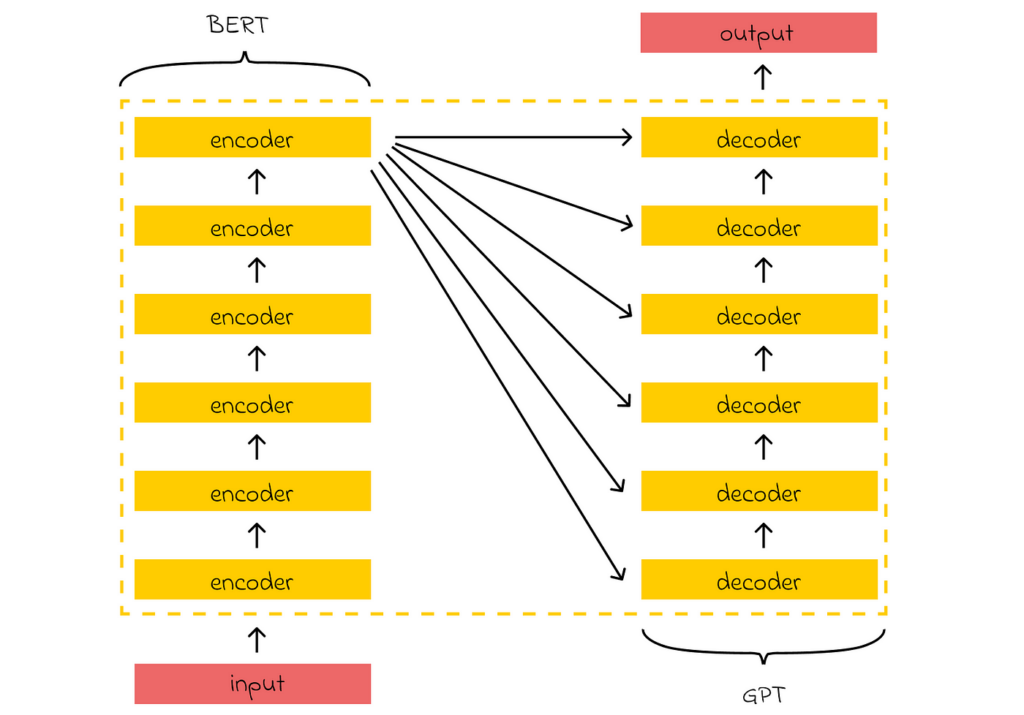
When it comes to LLMs, I strongly recommend studying the evolution of at least the first three GPT models, as they have had a significant impact on the AI world we know today. In particular, I would like to highlight the concepts of few-shot and zero-shot learning, introduced in GPT-2, which enable LLMs to solve text generation tasks without explicitly receiving any training examples for them.
Another important technique developed in recent years is retrieval-augmented generation (RAG). The main limitation of LLMs is that they are only aware of the context used during their training. As a result, they lack knowledge of any information beyond their training data.

The retriever converts the input prompt into an embedding, which is then used to query a vector database. The database returns the most relevant context based on the similarity to the embedding. This retrieved context is then combined with the original prompt and passed to a generative model. The model processes both the initial prompt and the additional context to generate a more informed and contextually accurate response.
A good example of this limitation is the first version of the ChatGPT model, which was trained on data up to the year 2022 and had no knowledge of events that occurred from 2023 onward.
To address this limitation, OpenAI researchers developed a RAG pipeline, which includes a constantly updated database containing new information from external sources. When ChatGPT is given a task that requires external knowledge, it queries the database to retrieve the most relevant context and integrates it into the final prompt sent to the machine learning model.
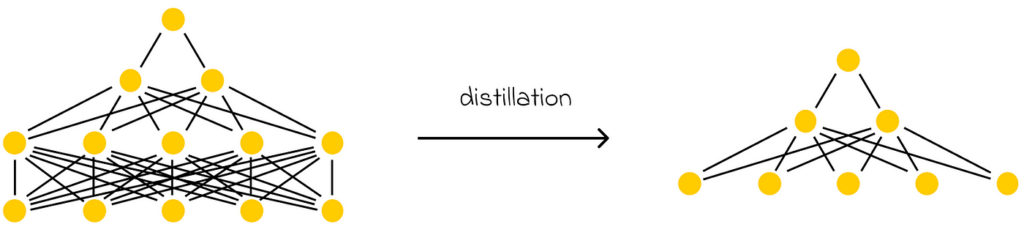
The goal of distillation is to create a smaller model that can imitate a larger one. In practice, this means that if a large model makes a prediction, the smaller model is expected to produce a similar result.
In the modern era, LLM development has led to models with millions or even billions of parameters. As a consequence, the overall size of these models may exceed the hardware limitations of standard computers or small portable devices, which come with many constraints.
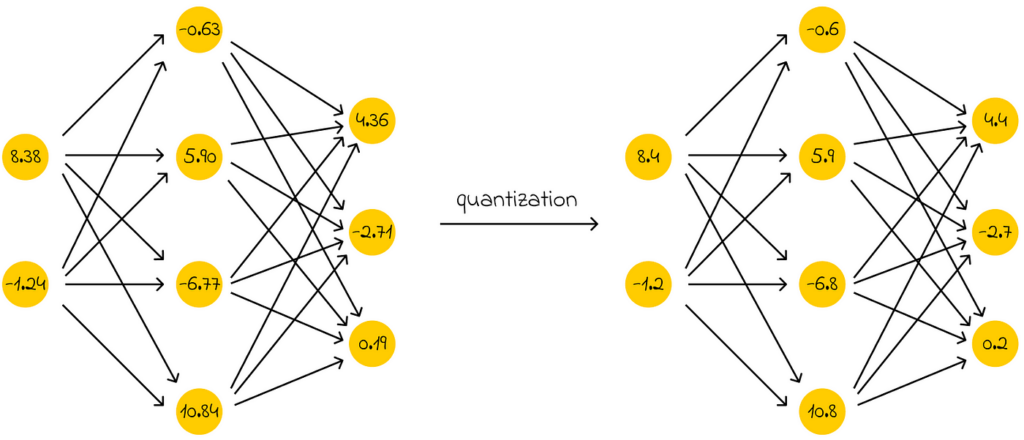
Quantization is the process of reducing the memory required to store numerical values representing a model’s weights.
This is where optimization techniques become particularly useful, allowing LLMs to be compressed without significantly compromising their performance. The most commonly used techniques today include distillation, quantization, and pruning.
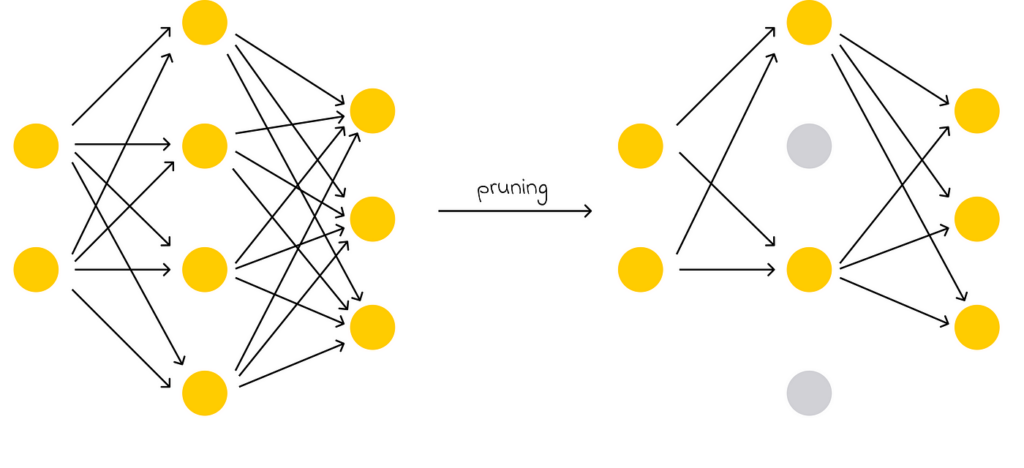
Pruning refers to discarding the least important weights of a model.
Fine-tuning
Regardless of the area in which you wish to specialize, knowledge of fine-tuning is a must-have skill! Fine-tuning is a powerful concept that allows you to efficiently adapt a pre-trained model to a new task.
Fine-tuning is especially useful when working with very large models. For example, imagine you want to use BERT to perform semantic analysis on a specific dataset. While BERT is trained on general data, it might not fully understand the context of your dataset. At the same time, training BERT from scratch for your specific task would require a massive amount of resources.
Here is where fine-tuning comes in: it involves taking a pre-trained BERT (or another model) and freezing some of its layers (usually those at the beginning). As a result, BERT is retrained, but this time only on the new dataset provided. Since BERT updates only a subset of its weights and the new dataset is likely much smaller than the original one BERT was trained on, fine-tuning becomes a very efficient technique for adapting BERT’s rich knowledge to a specific domain.
Fine-tuning is widely used not only in NLP but also across many other domains.
# 05. Computer vision
As the name suggests, computer vision (CV) involves analyzing images and videos using machine learning. The most common tasks include image classification, object detection, image segmentation, and generation.
Most CV algorithms are based on neural networks, so it is essential to understand how they work in detail. In particular, CV uses a special type of network called convolutional neural networks (CNNs). These are similar to fully connected networks, except that they typically begin with a set of specialized mathematical operations called convolutions.
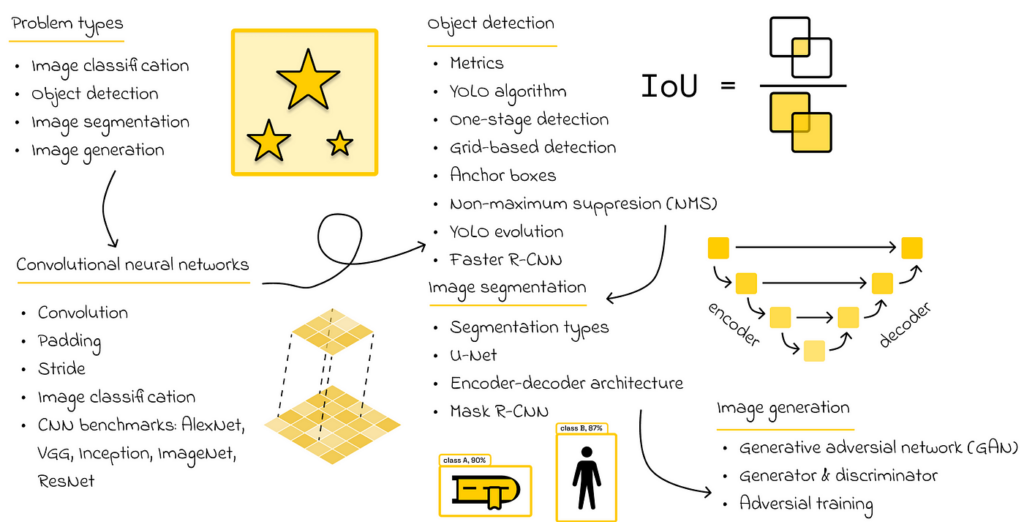
In simple terms, convolutions act as filters, enabling the model to extract the most important features from an image, which are then passed to fully connected layers for further analysis.
The next step is to study the most popular CNN architectures for classification tasks, such as AlexNet, VGG, Inception, ImageNet, and ResNet.
Speaking of the object detection task, the YOLO algorithm is a clear winner. It is not necessary to study all of the dozens of versions of YOLO. In reality, going through the original paper of the first YOLO should be sufficient to understand how a relatively difficult problem like object detection is elegantly transformed into both classification and regression problems. This approach in YOLO also provides a nice intuition on how more complex CV tasks can be reformulated in simpler terms.
While there are many architectures for performing image segmentation, I would strongly recommend learning about UNet, which introduces an encoder-decoder architecture.
Finally, image generation is probably one of the most challenging tasks in CV. Personally, I consider it an optional topic for learners, as it involves many advanced concepts. Nevertheless, gaining a high-level intuition of how generative adversial networks (GAN) function to generate images is a good way to broaden one’s horizons.
In some problems, the training data might not be enough to build a performant model. In such cases, the data augmentation technique is commonly used. It involves the artificial generation of training data from already existing data (images). By feeding the model more diverse data, it becomes capable of learning and recognizing more patterns.
# 06. Other areas
It would be very hard to present in detail the Roadmaps for all existing machine learning domains in a single article. That is why, in this section, I would like to briefly list and explain some of the other most popular areas in data science worth exploring.
First of all, recommender systems (RecSys) have gained a lot of popularity in recent years. They are increasingly implemented in online shops, social networks, and streaming services. The key idea of most algorithms is to take a large initial matrix of all users and items and decompose it into a product of several matrices in a way that associates every user and every item with a high-dimensional embedding. This approach is very flexible, as it then allows different types of comparison operations on embeddings to find the most relevant items for a given user. Moreover, it is much more rapid to perform analysis on small matrices rather than the original, which usually tends to have huge dimensions.
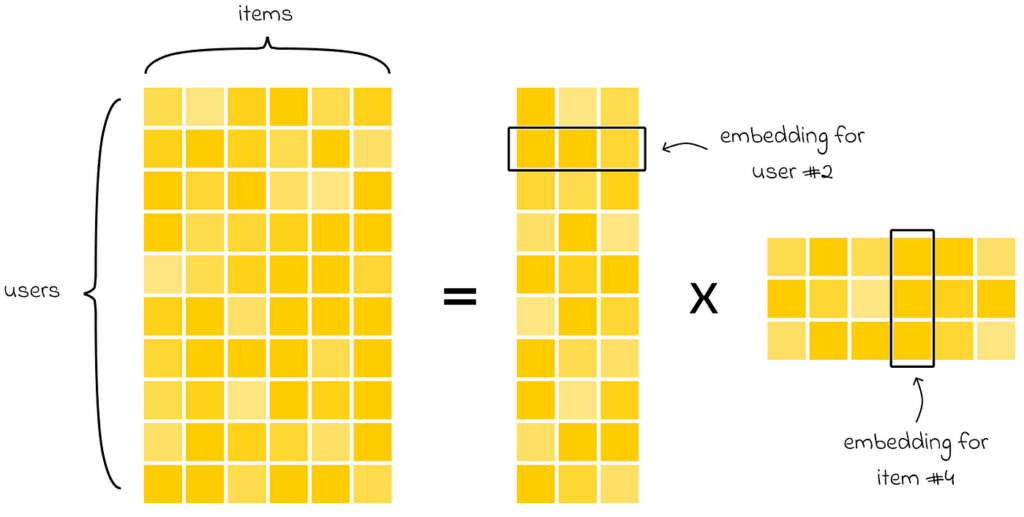
Ranking often goes hand in hand with RecSys. When a RecSys has identified a set of the most relevant items for the user, ranking algorithms are used to sort them to determine the order in which they will be shown or proposed to the user. A good example of their usage is search engines, which filter query results from top to bottom on a web page.
Closely related to ranking, there is also a matching problem that aims to optimally map objects from two sets, A and B, in a way that, on average, every object pair (a, b) is mapped “well” according to a matching criterion. A use case example might include distributing a group of students to different university disciplines, where the number of spots in each class is limited.
Clustering is an unsupervised machine learning task whose objective is to split a dataset into several regions (clusters), with each dataset object belonging to one of these clusters. The splitting criteria can vary depending on the task. Clustering is useful because it allows for grouping similar objects together. Moreover, further analysis can be applied to treat objects in each cluster separately.
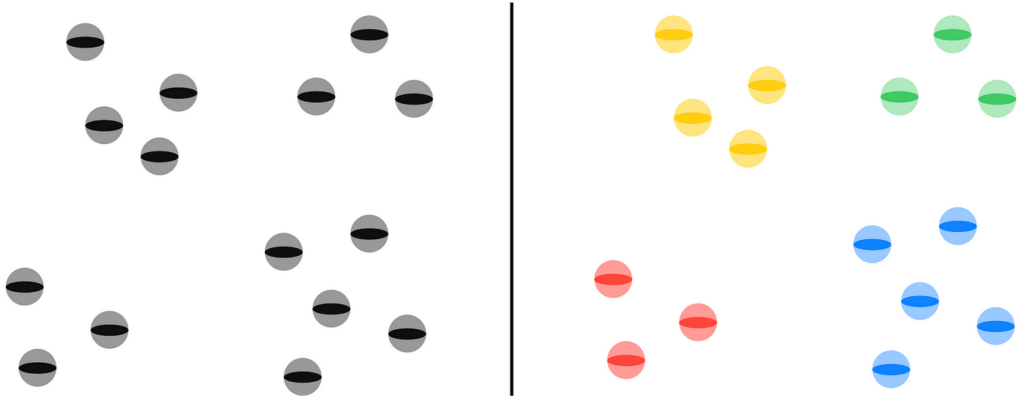
The goal of clustering is to group dataset objects (on the left) into several categories (on the right) based on their similarity.
Dimensionality reduction is another unsupervised problem, where the goal is to compress an input dataset. When the dimensionality of the dataset is large, it takes more time and resources for machine learning algorithms to analyze it. By identifying and removing noisy dataset features or those that do not provide much valuable information, the data analysis process becomes considerably easier.
Similarity search is an area that focuses on designing algorithms and data structures (indexes) to optimize searches in a large database of embeddings (vector database). More precisely, given an input embedding and a vector database, the goal is to approximately find the most similar embedding in the database relative to the input embedding.
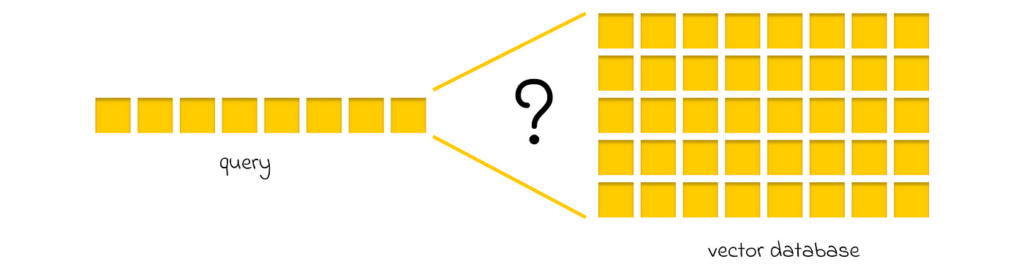
The goal of similarity search is to approximately find the most similar embedding in a vector database relative to a query embedding.
The word “approximately” means that the search is not guaranteed to be 100% precise. Nevertheless, this is the main idea behind similarity search algorithms — sacrificing a bit of accuracy in exchange for significant gains in prediction speed or data compression.
Time series analysis involves studying the behavior of a target variable over time. This problem can be solved using classical tabular algorithms. However, the presence of time introduces new factors that cannot be captured by standard algorithms. For instance:
- the target variable can have an overall trend, where in the long term its values increase or decrease (e.g., the average yearly temperature rising due to global warming).
- the target variable can have a seasonality which makes its values change based on the currently given period (e.g. temperature is lower in winter and higher in summer).
Most of the time series models take both of these factors into account. In general, time series models are mainly used a lot in financial, stock or demographic analysis.
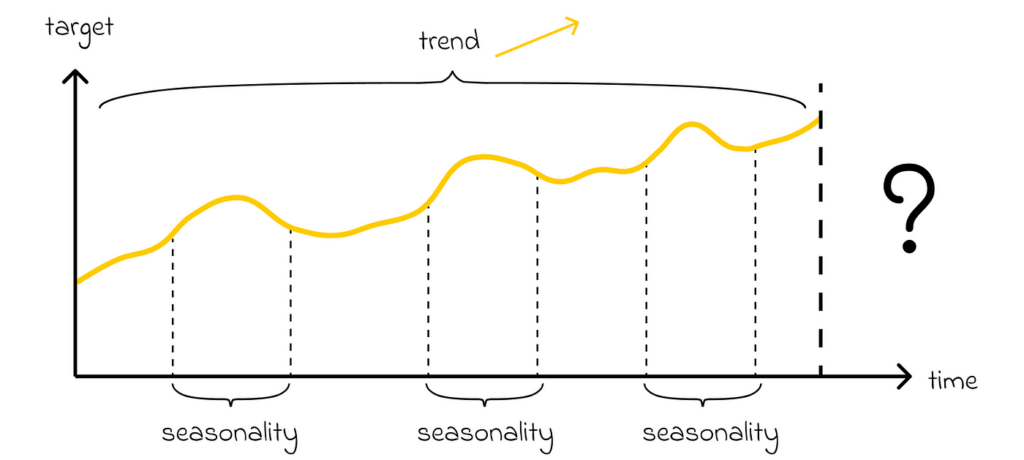
Another advanced area I would recommend exploring is reinforcement learning, which fundamentally changes the algorithm design compared to classical machine learning. In simple terms, its goal is to train an agent in an environment to make optimal decisions based on a reward system (also known as the “trial and error approach”). By taking an action, the agent receives a reward, which helps it understand whether the chosen action had a positive or negative effect. After that, the agent slightly adjusts its strategy, and the entire cycle repeats.
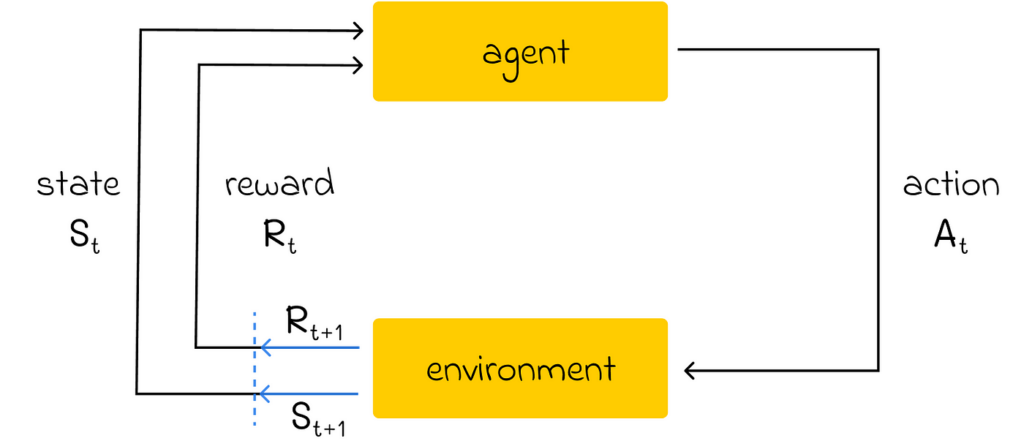
Reinforcement learning is particularly popular in complex environments where classical algorithms are not capable of solving a problem. Given the complexity of reinforcement learning algorithms and the computational resources they require, this area is not yet fully mature, but it has high potential to gain even more popularity in the future.

Currently the most popular applications are:
- Games. Existing approaches can design optimal game strategies and outperform humans. The most well-known examples are chess and Go.
- Robotics. Advanced algorithms can be incorporated into robots to help them move, carry objects or complete routine tasks at home.
- Autopilot. Reinforcement learning methods can be developed to automatically drive cars, control helicopters or drones.
Conclusion
This article was a logical continuation of the previous part and expanded the skill set needed to become a data scientist. While most of the mentioned topics require time to master, they can add significant value to your portfolio. This is especially true for the NLP and CV domains, which are in high demand today.
After reaching a high level of expertise in data science, it is still crucial to stay motivated and consistently push yourself to learn new topics and explore emerging algorithms.
Data science is a constantly evolving field, and in the coming years, we might witness the development of new state-of-the-art approaches that we could not have imagined in the past.
Resources
All images are by the author unless noted otherwise.